Recent News
Seminar: Maxwell D. Gregoire
December 5, 2024
Seminar: Adarsh Venkataramani
November 22, 2024
Seminar: Stavroula Foteinopoulou
November 14, 2024
Seminar: Mark Gilmore
November 8, 2024
News Archives
Jayaweera/Aref Win Best Paper at CROWNCOM
July 1, 2019
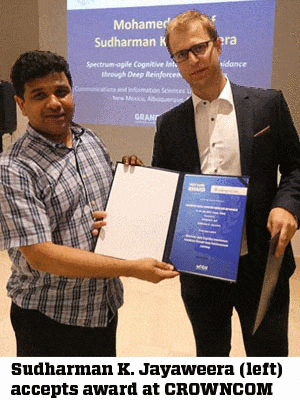
ECE Professor Sudharman Jayaweera accepted the best paper award at the 14th EAI Int'l Conference on Cognitive Radio Oriented Wireless Networks (CROWNCOM) held in Poznan, Poland on 11-12 June 2019.
The paper was entitled "Spectrum-agile Cognitive Interference Avoidance through Deep Reinforcement Learning" and was co-authored by ECE PhD student Mr Mohamed A. Aref, who is working in the Communications and Information Sciences lab (CISL) at the ECE department under the supervision of Dr. Jayaweera.
The main goal of CROWNCOM 2019 is to bring together researchers and present their new solutions of cognitive radio systems and their potential help with delivering required stringent requirements of future 5G and beyond 5G networks.
"In this work we proposed using deep reinforcement learning, a branch of deep learning, to enable radios to avoid interference and jamming signals," said Aref in a recent e-mail.
Aref has been a PhD student at ECE since Jan. 2016. He was involved in many important research projects that were funded by the Air Force Research Laboratory (AFRL) and the Army Research Laboratory (ARL). His research spans software-defined radios, cognitive radios, machine learning, and wireless communications.
CROWNCOM is organized by European Alliance for Innovation and Poznań University of Technology with Honorary Patronage of the Mayor of Poznań.
Here is an abstract of the paper:
One of the research trends nowadays is to investigate the applications of machine learning, especially deep learning, techniques in wireless communications domain. In the coming years, deep learning is expected to play an important role in future wireless communications networks design including Internet of Things (IoT) and the 6th generation (6G) cellular communication systems.
In this work we proposed using deep reinforcement learning, a branch of deep learning, to enable radios to avoid interference and jamming signals. This yields to a promising design for spectrum-agile smart radios, named wideband autonomous cognitive radios (WACRs). Recently, these radios become essential to achieve spectrum situational awareness and signals intelligence in many applications including aerospace, military, and homeland security.
One of the major advantages of the proposed cognitive interference avoidance approach is its ability to work in a partially observable wideband spectrum. Furthermore, it is compatible with both high and low-performance computing platforms. It is shown through simulations and experimental results that the proposed algorithm can rapidly reconfigure to tackle sudden changes in the RF environment making it suitable for real-time applications in heterogeneous environments.