ECE PhD Graduates
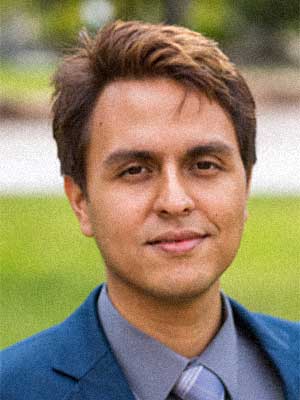
Jorge Canales Verdial [Spring 2023]
04-14-2023
Biography
Mr. Jorge Canales Verdial defended his Ph.D. Friday, April 14 in a meeting chaired by ECE Prof. Dr. Dr. Payman Zarkesh-Ha at CHTM in room 103.
Abstract
The data generated by spectroscopic sensors can be analyzed with minimal power consumption through the use of the neuromorphic architectures and hardware. Moreover, by implementing filamentary resistive random-access memory (ReRAM) arrays in the development of these ultra-low-power neuromorphic computing radionuclide detection systems, new computational avenues can be explored that utilizes memristor devices' intrinsic and inherent reconfigurable dynamics. In this work, small-scale functional prototypes using spiking sparse locally competitive approximations are tested for said applications. The demonstration hardware uses filamentary ReRAM arrays, while consuming minimal energy for radionuclide detection. The proposed systems perform exceptionally well in terms of accuracy and throughput. In light of these results, it is demonstrated that memristor-based computing is the most appropriate technology for developing energy-efficient radiation detection platforms.
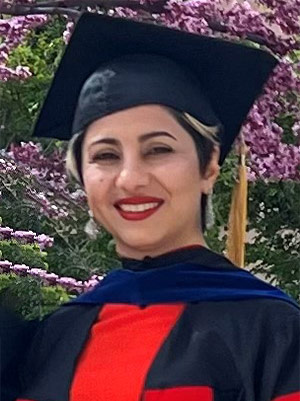
Zahra Abedi [Spring 2023]
04-13-2023
Biography
Ms. Zahra Abedi defended her Ph.D. at 9am on Thursday, April 13 in a Zoom meeting chaired by Dr. Edl Schamiloglu.
Abstract
This research addresses the issue of electromagnetic interference (EMI) in digital electronics, which can cause undesired behavior in active electronic components and systems. As technology advances and transistor size decreases, devices become more susceptible to EMI. In addition, as voltage is scaled down to save energy, silicon chips become more susceptible to soft errors. While there are several ways to mitigate the impact of severe EMI on digital systems, minimal electromagnetic coupling can still cause significant problems. We develop analytical models to predict device upset due to EMI.
The study focuses on identifying vulnerable parameters of operation related to device upsets under interference and investigating the correlation between upsets and interference characteristics, such as power and frequency, based on the device technology node. The predictive models are successfully compared against measurement data from devices that were fabricated using TSMC’s 180 nm, 130 nm, and 65 nm standard CMOS processes which confirms their effectiveness in accurately predicting the system’s behavior under low frequency RF injection.
We first explore the impact of EMI on the leakage current of ICs, which is a major source of power dissipation. We found that even a small amount of EMI at low frequency can drastically increase leakage current, causing battery drainage and temperature rise, while the device appears to be operating normally. For example, a 340 mV of peak noise (or 0.6 dBm) can increase the leakage current by factor of 1000. We develop an analytic model to predict the inverter’s leakage susceptibility as a function of the frequency and power of the injected EMI. The model successfully predicts the behavior of EMI injection on the leakage current in a CMOS inverter over a wide range of frequencies up to 4 GHz. As anticipated, the leakage current of the inverter increases as the amplitude of the RF signal increases. However, this change is relatively smaller at higher frequencies. Finally, we investigate the impact of RF injection on the eye height and signal-to-noise ratio (SNR) of a 10XINV, a fundamental element in digital circuits. The results show that RF injection causes significant degradation of the eye diagram, resulting in a reduction in the eye height and SNR values. For example, a peak noise of 251 mV (or -2 dBm) can lead to a nearly 50% reduction in eye height of 180 nm technology node.
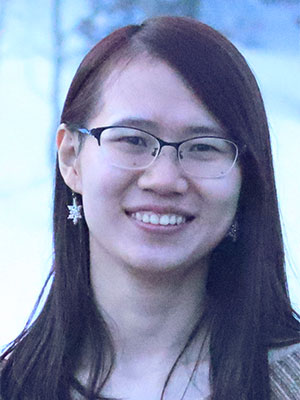
Wenjing Shi [Spring 2023]
04-10-2023
Biography
Abstract
The dissertation studies the problem of accessing student participation based on classroom videos. The development of effective participation methods requires ro-bust solutions to human recognition, student group detection, and long-term tracking under occlusion, variation of camera angles, and sitting arrangements in actual class-room environments. Ultimately, the goal of the research is to support educational researchers in properly assessing how students participate in classroom activities.
The dissertation develops new methods for assessing student participation in long (>1 hour) classroom videos. First, the dissertation introduces the use of multiple image representations based on raw RGB images and AM-FM components to solve the group detection problem. Second, a dynamic scene analysis model is developed for tracking under occlusion and variable camera angles. Third, a motion vector projection system is used for identifying instances of students talking.
The approaches are validated using digital videos from the Advancing Out-of-school Learning in Mathematics and Engineering (AOLME) project. The methods are used to create activity maps to visualize and quantify students' presence in each session. The dataset contains several hours-long videos from multiple sessions. For group detection, the method was tested with 13 long videos. Compared with the current state-of-the-art methods, the proposed method provides a better group detection result by filtering out the background groups. For talking detection, for four long videos, the method achieved an accuracy of 59% compared to 42% for TSN and 45% for C3D. For dynamic participant tracking, the proposed method achieves 99.2% accuracy on a test set of short videos (10 to 150 seconds). On a test set of 6 long videos (23 minutes 45 seconds), the dynamic scene analysis method achieved an average accuracy of 84.1% compared to 61.9% based on static analysis.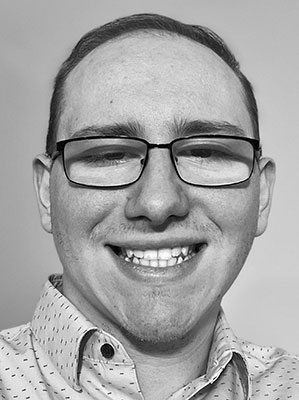
Shawn Priore [Spring 2023]
04-07-2023
Biography
Mr Shawn Priore defended his PhD Dissertation on Friday, April 7 at 2pm in ECE 125E in a meeting chaired by Dr Meeko Oishi.Abstract
Stochastic disturbances arise in a variety of engineering applications. For tractability, Gaussian disturbances are often assumed. However, this may not always be valid, such as when a disturbance exhibits heavy-tailed or skewed phenomena. As autonomous systems become more ubiquitous, non-Gaussian disturbances will become more common due to the compounding effects of sensing, actuation, and external forces. Despite this, little has been done to develop formal methods that are both computationally efficient and allow for analytical assurances with non-Gaussian disturbances. Addressing convex polytopic set acquisition and non-convex collision avoidance chance constraints with quantile and moment-based reformulations, this dissertation proposes novel stochastic optimal control techniques that are computationally efficient and allow for analytic guarantees with arbitrary disturbances. These reformulations are amenable to optimization techniques while eliminating costly, and frequently intractable, high-dimensional integrals. These conservative reformulations guarantee chance constraint satisfaction and are numerically tractable. I demonstrate these methods with applications to multi-satellite operations.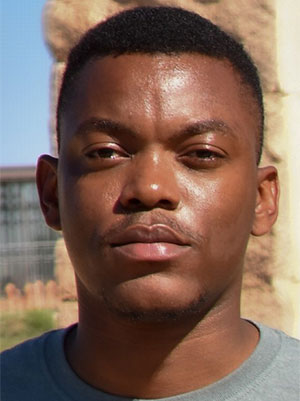
Fisayo Sangoleye [Spring 2023]
04-06-2023
Biography
Mr. Fisayo Sangoleye defend his PhD Dissertation on Thursday, April 6 at 9 am in a Zoom meeting chaired by Dr Eirini Eleni Tsiropoulou.
Abstract
Cyber-physical systems (CPS) transform how humans interact with technology by integrating sensing, computation, networking, and control with physical processes in order to facilitate smart services and innovative applications in our environments. Recent advances in CPS have led to rapid growth in the amount of information being constantly generated by people, systems, and processes. Most of this information, however, is underutilized due to the lack of efficient information utilization and decision-making techniques. Also, the increasing interconnectivity of CPSs present security risks that, if left unaddressed, could be highly disruptive to systems, processes and economies. In this dissertation, we present a study and proposal of novel solutions to the problems of security and decision-making in Cyber-physical systems using advanced theoretical concepts such as Reinforcement Learning and Contract Theory.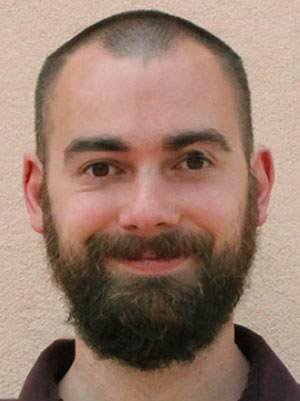
Adam Thorpe [Spring 2023]
03-31-2023
Biography
Mr. Adam Thorp defended his PhD Dissertation on Friday (March 31) at 1 pm in a meeting chaired by Dr Meeko Oishi in ECE 118Abstract
Autonomous systems are increasingly being deployed in complex environments subject to real-world uncertainty. For such systems, it may be exceptionally difficult or even impossible to compute a simple mathematical model of the system --- for instance due to the presence of human elements, complex mechanics of system dynamics, or learning-enabled components. Data-driven control has recently gained significant attention in this area, where observations taken from the system evolution are used to compute an implicit representation of the system that is amenable to analysis and control. However, data-driven algorithms for control present new challenges, and require new insights to enable their use.
The primary focus of this thesis are algorithms for data-driven control based in the theory of reproducing kernal Hilbert spaces. Specifically, we focus on using data to compute empirical estimates of key statistical quantities in stochastic optimal control problems based in the framework of kernel embeddings of distributions.
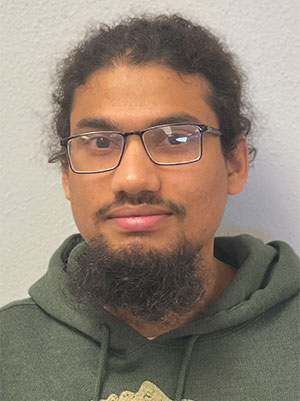
Nafis Irtija [Spring 2023]
03-31-2023
Biography
Mr Nafis Irtija defended his PhD Dissertation in a Zoom Meeting held on Friday, March 31) at 11 am in a Zoom meeting chaired by. Dr Eirini Eleni Tsiropoulou.
Abstract
There has been an increasing need for an adequate control system that can seamlessly combine the various components of the evolving quantum computing systems. The most common way of performing quantum gates in a trapped ion quantum computer uses modulated laser pulses that interact with the ion qubits. These pulses are coherently controlled using radiofrequency signals that modulate their timing, frequency, phase, and amplitude. In this dissertation, we focus our research on the design of a very low-latency and high-throughput control system for trapped ion quantum computing systems based on the System-on-a-chip technology. Towards this goal, we have designed an architecture combining an application processing unit, a real-time processing unit and programmable logic fabric of FPGAs. We have designed the system to achieve the maximum achievable performance possible, and we have measured the performances of all the interconnects extensively. Additionally, we present our work on an energy-efficient edge computing solution enabled by the Satisfaction Games Approximate Computing. Specifically, we utilize a Heterogeneous Approximate DNN accelerator to support an IoT environment by exploiting the Mobile Edge Computing technology and the Fully Autonomous Aerial Systems.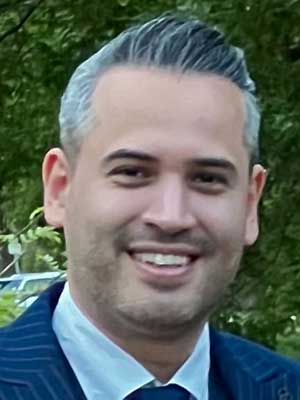
Seyyed Ali Ghorashi Khalil Abad [Spring 2023]
03-30-2023
Biography
Mr. Seyyed Ali Ghorashi Khalil Abadi will defend his PhD dissertation “Effective utilization of battery-supercapacitor hybrid energy storage systems in DC nano-grids” on Thursday, March 30 at 3 pm in a Zoom meeting chaired by Dr. Ali Bidram.Abstract
This PhD dissertation proposes novel control strategies to improve the flexibility and reliability of DC nanogrids by utilizing battery-supercapacitor hybrid energy storage systems (HESSs). The dissertation is divided into five sections, each addressing a specific challenge in DC nanogrids and proposing unique control strategies to overcome them. The proposed strategies include an adaptive control system for multiple nanogrids, a distributed power management strategy for a grid-connected DC nano-grid, a model predictive control strategy to improve voltage and stability in islanded DC nanogrids, a cloud HESS technology to improve voltage stability in clustered DC nanogrids, and a method of electric vehicle (EV) charging to utilize internal supercapacitors to improve battery lifetime and voltage quality of DC nanogrids. The proposed methods aim to improve power quality, flexibility, and efficiency of DC nanogrids, reduce power fluctuations, and enhance the lifetime of battery energy storage systems.
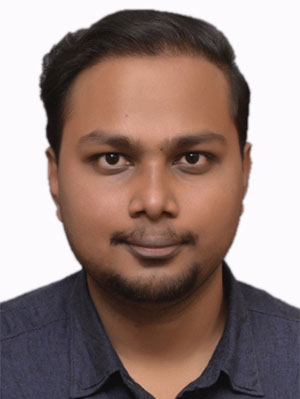
Rahul Jaiswal [Spring 2023]
03-17-2023
Biography
Mr Rahul Jaiswal will defend his dissertation, "Machine learning based prediction models for silicon heterojunction solar cell optimization" on Friday, March 17 at 9 am in a Zoom meeting chaired by Dr. Tito Busani.
Abstract
Silicon heterojunction solar cell of Heterojunction with Thin Intrinsic Layer (HIT) structure is a commercially available technology, and its market share will significantly increase by the next decade. With such a significant market share, any minor improvement in the device's overall efficiency can be beneficial three folds --- customer return on investment, industry revenue, and the overall carbon footprint (from manufacturing to recycling/disposing of the device). Conventionally, device optimization for solar cells has been achieved using a hit & trial approach where multiple experiments are done to evaluate the best process conditions and device parameters. This approach has some inherent disadvantages, especially, because it is expensive in terms of resources, time and manpower required. In the past couple of decades, simulation techniques are also being utilized in addition to the conventional approaches and very recently use of data science-based techniques has become popular in research and is gaining some traction in the photovoltaics industry.
In this doctoral research, an innovative approach is presented, where device simulations for solar cells are designed and calibrated to match the performance of an industrially manufactured device using a minimal set of measurement data. Then a digital twin for the numerical simulations is developed by training Machine Learning (ML) models using simulation and measurement data. ML methods are also used for the calibration of simulation models in addition to being used for the digital twin. The use of machine learning helps significantly reduce computational time ot prototype any design changes in the device. In our work, apart from providing mean predictions, we are also providing a measure of uncertainty with every prediction, using Gaussian Process Regression (GPR).
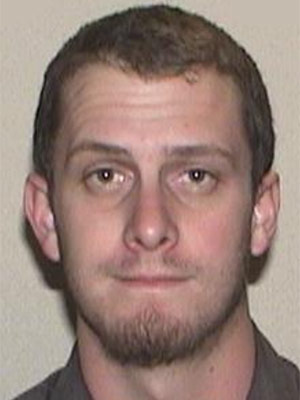
Troy Powell [Spring 2023]
01-25-2023
Biography
Troy Powell defended his dissertation "Theory, Simulation, and Experiments on a Magnetically Insulated Transmission Line Terminated by a Bremsstrahlung Diode" in a meeting chaired by Dr. Edl Schamiloglu on Wed, January 25 at 2 pm.Abstract
The study aims in applying combinatorial structures motivated from the basic CNN in various applications. Convolutional neural networks (CNNs) have grown to be very popular in the field of deep learning. The ability of such networks to learn both Spatial and temporal characteristics in the data have helped in deploying them in various fields. Through this research we explore different kinds of CNN-derived architectures and how these structures can be trained and setup in combinatorial environment to solve prob- lems in deep learning applications.
First, we introduce the basic idea of a deep learning meta-structures which is used in the application of facial expression recognition. Next, we investigate CNN meta-structures in detail in the application of Super-resolution. Further, the analysis of different combinatorial models containing CNN meta-structures and machine learning approaches are studied in detail for two different applications.
The first application is a medical imaging application for detecting Malarial retinopathy with high specificity and second is for developing an adaptive protection scheme to detect faults and topology change in distribution systems. Overall, the proposed work investigates the analysis of meta-structures derived from basic CNNs arranged in different combinations.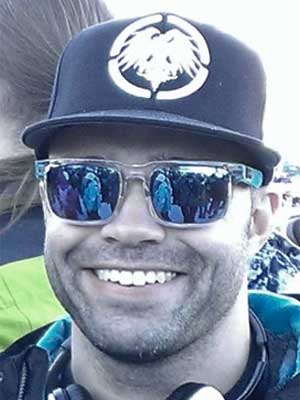
Kevin Shipman [Fall 2022]
12-16-2022
Biography
Mr. Kevin Shipman defended his dissertation, "Particle-in-Cell Simulations of Plasma Sheath Effects on a Whistler Wave Antenna Operating in the Inner Magnetosphere”" before Dr. Mark Gilman and his committee in a Zoom meeting that took place on December 16, 2022 .
Abstract
This abstract will be published pending a declassification process that is currently in progress.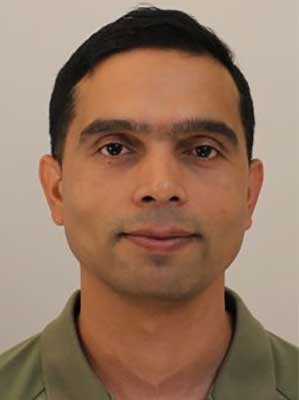
Binod Poudel [Fall 2022]
11-11-2022
Biography
Mr. Binod Poudel defended his PhD dissertation in a Zoom meeting chaired by ECE Prof Ali Bidram on November 11 at 1 p.m.Abstract
Modernizing power grids by communication-based technologies has introduced new challenges to the operation of grid specially when communication network experiences failure, or data is corrupted during the transfer. This issue is studied in this dissertation from the control and protection perspective. First, the cyber attack detection and mitigation of distributed control of microgrids is addressed when the distributed energy resources (DER) are exposed to false data injection attack. A cyber-threat detection technique is proposed based on Kullback-Liebler divergencebased criterion. This criterion with a threshold can detect the misbehaviour of a compromised DER control unit and, consequently calculates the interior-belief factor and communicates it with its neighboring DERs to inform them of the reliability of its outgoing information. From the protection point of view, the focus is on the communication failure of adaptive protection systems which highly rely on the communication network. To address the communication failure of adaptive protection system, this dissertation proposes a local adaptive modular protection (LAMP) architecture. A LMAP unit provides an adaptive, setting less, and communication free protection. Using the locally measured information of voltages and currents, this module can find the circuit topology using machine learning techniques without any information from other parts of distribution system. After finding the topology, each LAMP uses machine learning algorithms for identifying fault type. Moreover, when a fault occurs in the system, a proper coordination between relays is necessary. This can be achieved using the zone classification. During zone classification, machine learning algorithms are used to find the fault zone and location. This dissertation has verified the validity of the proposed approaches using simulation of different test circuits in different commercially available software packages.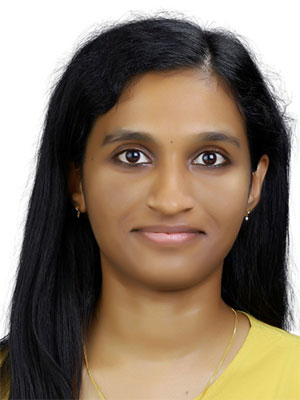
Meenu Ajith [Summer 2022]
07-11-2022
Biography
Ms. Meenu Ajith defended her dissertation, " Exploratory Analysis of Machine Learning for Images: Methods and Applications " before Dr. Manel Martinez-Ramon and his committee on Monday, July 11th at 11 am in ECE 237.Abstract
This research focuses on implementing four different applications of machine learning on images. The various categories of digital images considered for these applications are grayscale, RGB, and infra-red images. The first framework uses an unsupervised learning strategy for detecting fire and smoke from an infra-red image dataset. This problem was solved using a classical machine learning algorithm since the dataset was small and unlabeled. Next, a semi-supervised deep learning model was used for facial expression recognition. Here we detect emotions from a moderately large dataset containing labeled and unlabeled grayscale images. The third application focused on single image super- resolution, which was developed to increase the resolution of the input RGB image. The approach used deep learning due to the availability of sufficiently large, labeled data. The final structure consisted of a multimodal deep learning-based system for solar irradiance forecasting for cloudy days. The data used for these former applications consists of only images, whereas this work uses multimodal data, i.e., time-series data and infra-red images. Therefore, this research aims to consolidate heterogeneous, disconnected data from various sources to produce robust predictions. The performance evaluations of all the algorithms were done by comparing them with the state-of-the-art methods, and it was found that the proposed methods outperform the standard models.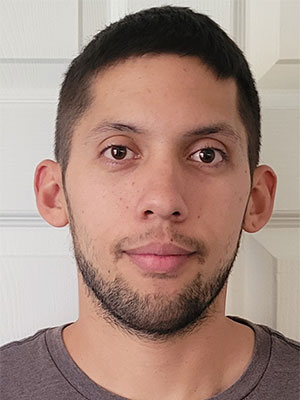
Justin Smith [Fall 2022]
10-28-2022
Biography
Mr. Justin K. Smith defended his PhD dissertation on Friday, Oct 28 at 9am in a meeting chaired by ECE Prof Jane Lehr, that took place in room 118 of the ECE Building.
Abstract
In present photoionization models used in computational studies, there is a lack of experimental data and several assumptions pertaining to photo-electron production in the gas. It is widely accepted that wavelengths between 98 nm and 102.5 nm are the primary contributors to photoionization of oxygen in an air plasma. In air, the collisional kinetics of nitrogen provides the photons, which results in the ionization of oxygen. Photoionization rate measurements from a low current corona discharge in synthetic air mixtures are reported to provide new empirical data. In addition, this provides a method to estimate the scaling parameter ω⁄α. Concurrently, vacuum ultraviolet emission (VUV) emission spectra from a nitrogen corona discharge at pressures from 0.1 – 60 Torr in the wavelength region of 95-105nm is reported. Identification of the bands reveal the transitions that are photoionizing capable.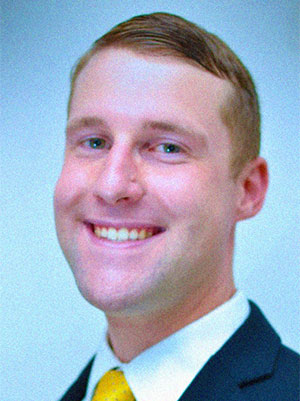
Michael Sherburne [Fall 2022]
11-07-2022
Biography
Mr. Michael Sherburne defended his Ph.D. at 12:30 on Monday (Nov 7) in a meeting chaired by ECE Prof. Dr. Edl Schamiloglu.Abstract
Location and Abstract Closed to the public for security reasons.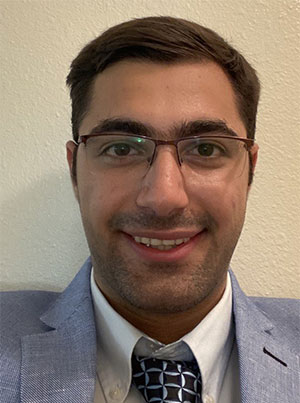
Tohid Khalili [Fall 2022]
11-04-2022
Biography
Mr. Tohid Khalili defended his PhD dissertation "Optimal Management of Renewable Energy Resources to Increase Resilience of Power Systems" in a Zoom meeting chaired by ECE Prof. Dr. Ali Bidram on Friday, November 4.Abstract
The main goal of this dissertation is to improve the resilience of power systems using different techniques and optimization approaches with regard to new technologies used in the existing power systems. In order to reach this goal, several test cases with various objective functions are proposed. Furthermore, the implementation of the demand response program is assessed with regard to risk factors in the microgrids to increase the profit of the system considering the uncertainty in the output of the renewable energy resources. Voltage regulation is also used to investigate and improve the system's financial and reliability status. In addition, COVID-19's effect with the high presence of renewable energy resources is examined from financial and reliability points of view in a multi-microgrid system. Finally, a new model for the wildfire risk is proposed in a large distribution system to increase the reliability of the system with the usage of reclosers and fuses with different schemes on one hand and reduce the newly presented wildfire risk index, on the other hand, using a heuristic algorithm to optimize the proposed model. The investigated topics address the resilience of electric power grids considering the optimization of different assets like placement and dispatching of renewable energy resources and locating protection devices.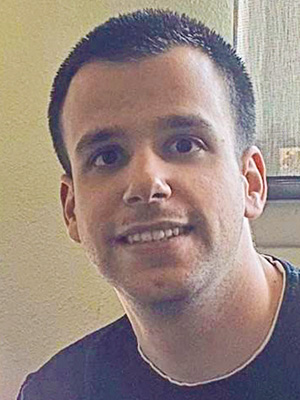
Georgios Fragkos [Fall 2022]
07-29-2022
Biography
Mr. Georgios Fragkos defended his dissertation, "Autonomous Decision Making in Interdependent Computing Systems based on Artificial Intelligence" on Friday, July 29 at 9 am in a Zoom Meeting chaired by Dr. Eirini Eleni Tsiropoulou.Abstract
With the advent of Artificial Intelligence (AI), the notion of autonomy, in terms of acting and thinking based on personal experience and judgment, has paved the way toward an autonomous decision-making future. This future can address the complex domain of the interdependent computing systems, whose main challenge is that they interact with each other with unpredictable and often unstable outcomes. It is crucial to envision and design this AI-driven autonomy for the reciprocal computing systems which cover a variety of use-cases ranging from the Internet of Things (IoT) to cybersecurity. This can be achieved by cloning the human decision-making process, which imposes that before humans decide how to act, they sense their unknown and stochastic environment, perform actions, and finally assess their perceived feedback. The feedback is subjectively evaluated as satisfactory or not by each human based on her personal behavioral profile and reasoning. The repetitive iteration of the aforementioned steps constitutes the learning process of humans. Consequently, the core idea is to inject human cognizance into the interdependent computing systems to transform them into AI-enabled decision-making agents who mimic the rational behavioral attributes of humans and optimize their subjective criteria autonomously. The rapid growth of interdependent computing systems, such as Unmanned Aerial Vehicles (UAVs) or Multi-Access Edge Computing servers (MEC), results in huge amounts of data and strict Quality of Service (QoS) requirements. When these systems act in an autonomous manner, they reveal a competitive behavior since each system aims at optimizing its own subjective criteria selfishly. This introduces the concept of interactive decision-making in non-cooperative environments, where the feedback for each system depends on the potentially conflicting actions of the rest. Therefore, we utilize Game Theory to efficiently capture these strategic interactions among the interdependent computing systems within the non-cooperative environments and prove that there exist solutions, i.e., stable Equilibrium points. The Equilibrium points are considered stable solutions because each system does not have a strategic incentive to change its own action unilaterally. To approach these Equilibria in a distributed manner we deploy Reinforcement Learning (RL), which enables the autonomous interdependent computing systems to be intelligent and learn in a stochastic environment by trial and error using the feedback from their own actions and experiences. Furthermore, the traditional RL methodology is enriched with the technique of reward reshaping to consider the Labor Economics-like arrangements among the autonomous interdependent computing systems via Contract Theory as well as their behavioral profiles via a Bayesian belief model.
The concurrent utilization of Game Theory and Reinforcement Learning with reward reshaping is a step toward Self-Aware Artificial Intelligence (SAAI). We prove that it has a great potential to be the main component for building autonomous decision-making interdependent computing systems based on AI and can be effectively utilized in various application domains.
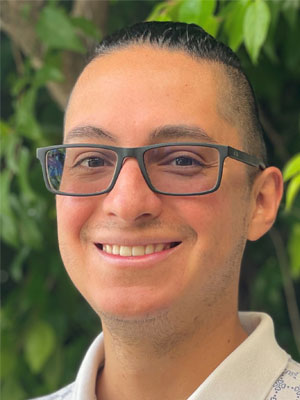
Jorge Diaz Cruz [Fall 2022]
08-02-2022
Biography
Mr. Jorge Diaz Cruz defended his dissertation, "Machine Learning for Control of Superconducting RF Cavities" before Dr. Edl Schamiloglu and his committee on Tuesday (August 2) at 9 am in ECE 118.Abstract
Linear Accelerator (LINAC) up- grade from a normal-conducting copper-based linac that is the original LCLS, in operations since 2009. This upgrade will permit higher repetition rates of the electron beams that are accelerated and then forced to radiate short-wavelength radiation throguh the FEL emission process. The quality of the X-rays produced by this machine depends on the quality of the electron beam. The new LINAC is composed of 35 cryomodule (CM)s, with 8 Superconducting RF (SRF) cavities per CM, and will accelerate electrons to an energy of 4 GeV with a continuous bunch repetition rate of up to 1 MHz, whereas the copper LINAC could only operate up to 120 Hz. The acceleration mode in such a TESLA-type SRF cavity in the LCLS-II is the π-mode of the first monopole passband at 1.3 GHz; however, higher frequency modes known as Higher Order Modes (HOM), which are proportional to the beam offset with respect to the electrical axis of the cavities, can also be excited producing unwanted beam emittance degradation. The energy injection into the first CM is <1 MeV, making the beam susceptible to emittance dilution effects due to wakefield kicks and off-axis beam transport. In this dissertation, we explore a beam offset monitor based on HOM signals using Machine Learning (ML) techniques. Moreover, the stability of the RF field inside the cavities directly impacts the quality of the accelerated electron beam. To control the cavity’s RF field amplitude and phase stability, a digital Low Level RF (LLRF) system implemented in a Field-programmable Gate Array (FPGA) was previously designed based on two Proportional-Integral (PI) controllers. We also explore a ML-based tuning of the PI gains for the amplitude and phase feedback loops of the LLRF system. Furthermore, due to the high loaded quality factor QL of the SRF cavities in the order of 4 × 107, the cavity bandwidth is 32 Hz. This imposes a requirement on cavity peak detuning of no more than 10 Hz to stay within the power budget of 3.8 KW. Continuous Wave (CW) superconducting machines with low beam loading are susceptible to microphonics-induced detuning, which can exceed the 10 Hz limit.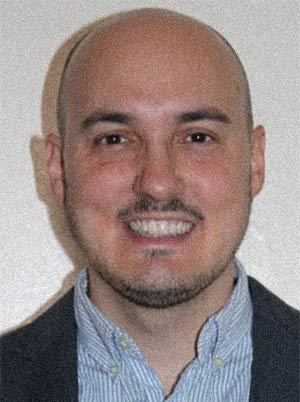
Miguel Angel Hombrados-Herrera [Summer 2022]
08-11-2022
Biography
Mr. Miguel Hombrados Herrera defended his dissertation in a Zoom meeting chaired by Dr. Manel Martinez-Ramon on Monday (April 11) at 11 am.
Abstract
The elevated costs that incur power grid stakeholders due to forecasting errors in power load demand have created the need for forecasting methods that provide ac- curate predictions and allow for assessing the reliability of their predictions. This thesis proposes a probabilistic forecasting method for multi-step ahead forecasting.
In particular, it presents a probabilistic method to perform a 24-hours-ahead power load forecasting that arises as the combination of Gaussian Process regressors with NMF (nonnegative matrix factorization) and integrates the advantages of both methods. Instead of training 24 independent processes for each hour of the predicted day, this work proposes to factorize the 24 hours power profile as the additive composition of a reduced number of K latent components estimated with K independent Gaussian Processes. This reduces considerably the training time invested in the regressors and introduces some interpretability to the model. The proposed method is compared with models built exclusively with Gaussian Processes in a state-of-the-art data set of aggregated load.
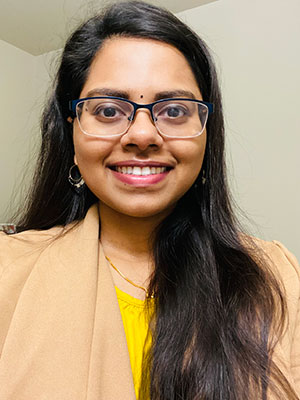
Aswathy Rajendra-Kurup [Summer 2022]
07-07-2022
Biography
Rajendra Kurup defended her dissertation "Combinatorial CNN meta-structures in deep learning applications" in an open Zoom meeting chaired by Dr. Manel Martinez-Ramon on Wed, July 7 at 11 am.Abstract
The study aims in applying combinatorial structures motivated from the basic CNN in various applications. Convolutional neural networks (CNNs) have grown to be very popular in the field of deep learning. The ability of such networks to learn both Spatial and temporal characteristics in the data have helped in deploying them in various fields. Through this research we explore different kinds of CNN-derived architectures and how these structures can be trained and setup in combinatorial environment to solve prob- lems in deep learning applications.
First, we introduce the basic idea of a deep learning meta-structures which is used in the application of facial expression recognition. Next, we investigate CNN meta-structures in detail in the application of Super-resolution. Further, the analysis of different combinatorial models containing CNN meta-structures and machine learning approaches are studied in detail for two different applications.
The first application is a medical imaging application for detecting Malarial retinopathy with high specificity and second is for developing an adaptive protection scheme to detect faults and topology change in distribution systems. Overall, the proposed work investigates the analysis of meta-structures derived from basic CNNs arranged in different combinations.
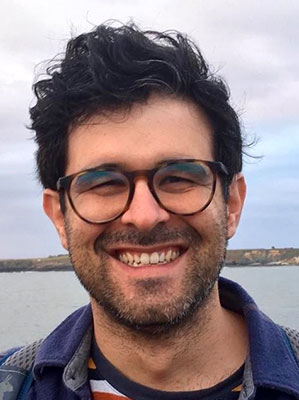
Guillermo Terren Serrano [Spring 2022]
03-31-2022
Biography
Guillermo Terren Serrano defended his Dissertation, "Intra-Hour Solar Forecasting using Cloud Dynamics Features Extracted from Ground-Based Infrared Sky Images" in a meeting chaired by Dr. Manel Martínez-Ramón at 3 pm on Thursday, March 31 in room 118 of ECE.Abstract
Due to the increasing use of photovoltaic systems, power grids are vulnerable to the projection of shadows from moving clouds. An intra-hour solar forecast provides power grids with the capability of automatically controlling the dispatch of energy, reducing the additional cost for a guaranteed, reliable supply of energy (i.e., energy storage). This dissertation introduces a novel sky imager consisting of a long-wave radiometric infrared camera and a visible light camera with a fisheye lens. The imager is mounted on a solar tracker to maintain the Sun in the center of the images throughout the day, reducing the scattering effect produced by the Sun's direct radiation. Features of the cloud dynamics are analyzed to compute the probability of the Sun intercepting air parcels in the sky images. Probabilistic and deterministic multi-task intra-hour solar forecasting algorithms are introduced, based on kernel and deep learning methods, to increase the penetration of photovoltaic systems in power grids.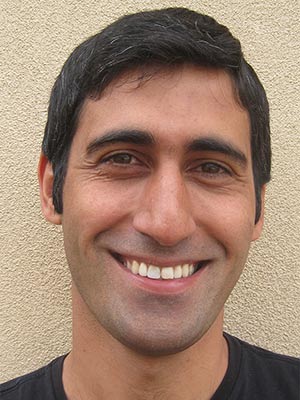
Amir Nafchi [Spring 2022]
03-30-2022
Biography
Amir Nafchi defended his dissertation "Multicarrier Modulation Using Discrete Fractional Fourier Transform" in a meeting chaired by Dr. Ramiro Jordan at 1 pm in Centennial Room 1030.Abstract
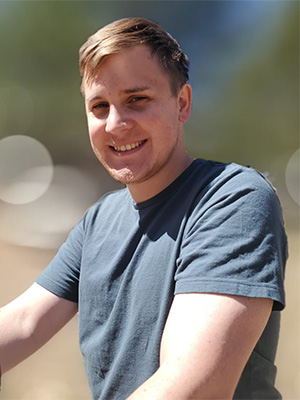
Artem Kuskov [Spring 2022]
02-25-2022
Biography
Artem Koskov defended his disseration, "Relativistic Magnetron with Diffraction Output and Split Cathode" in a hybrid Zoom meeting and ECE118, chaired by Dr. Edl Schamiloglu.
Abstract
The relativistic magnetron with diffraction output (MDO) is one of the most efficient microwave sources for sub-100 ns pulses. For pulsewidths greater than 100 ns, the microwave production stops due to anode-cathode (A − K) gap closure. In the pres-ence of a solid cathode, the anode and cathode plasmas expand towards each other, shorting the gap. A research team at The Technion Israel Institute of Technology proposed to replace a solid cathode with a split cathode in a magnetron with radial extraction to mitigate gap closure and maximize microwave production from a long pulse generator. Such a cathode geometry comprises an emitter (which injects the beam from outside the magnetron interaction region) and a reflector (downstream of the magnetron interaction region) at the same potential as the emitter.
This dissertation describes the problem and provides a detailed analysis of the split cathode behavior in a relativistic MDO.
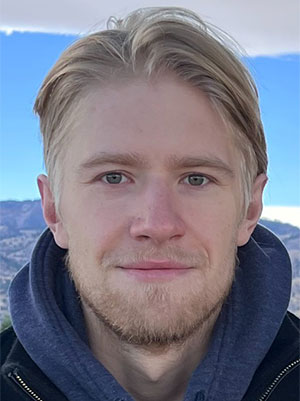
Dmitrii Andreev [Spring 2022]
04-08-2022
Biography
Mr. Dmitrii Andreev Heeger defended his PhD thesis on Friday, April 8 in a Zoom meeting chaired by Dr. Edl Schamiloglu. The title of Mr. Heeger's dissertation was "Relativistic Magnetron Powered by Electrons in a High-Energy State."
Abstract
A traditional relativistic magnetron is an efficient, high-power, widely adopted, and extensively studied source of cm-wave radiation. Recent advances demonstrated
GW-class microwave bursts of short 50-100 ns duration at 50% efficiency. Typically, a cathode sits inside the interaction region and produces an effective source of electrons to power the magnetron. However, the cathode plasma generated during explosive electron emission expands radially, leading to unwanted frequency drift and pulse shortening. Pulse shortening is a premature collapse of microwave oscillations that limits the total output energy per pulse. Additionally, the cathode and the anode suffer from electron bombardment, which limits their lifetime.
Another problem with relativistic magnetrons is electron leakage from the interaction region. Previously, magnetic endcaps were proposed for a magnetron with diffraction output (MDO) which reached 700 MW at 63% efficiency. However, the electric breakdown of the endcap occurred, and the efficiency dropped to 35%.
Several researchers proposed to move the cathode upstream of the interaction region and form a low-energy state of an electron beam by different means, such as manipulation of the vacuum tube radii and shaping the magnetic field into a magnetic mirror. These techniques were tested numerically or experimentally in this dissertation.
Recently, researchers at Technion, in collaboration with UNM, proposed a split cathode to form a low-energy "squeezed" state by injecting electrons into a potential well and squeezing them with a sufficiently strong magnetic field. A potential well is formed by two electrodes connected with a thin rod - a cathode outside of the interaction region and a downstream reflector. Experiments at Technion achieved a low-energy state with a split cathode, at output power and efficiency comparable to a physical cathode in the interaction region. Additionally, the split-cathode-driven magnetron demonstrated a longer pulse and smaller frequency drift than a cathode in the interaction region. Finally, the downstream reflector suppressed the leakage current and did not experience an electric breakdown. These advantages inspired numerical and experimental investigation of the split cathode at UNM.
This research aims to compare the output power and efficiency of solid and split cathodes and to confirm the suppression of leakage current. Additionally, it aims to answer if the split cathode-driven magnetron operates like a traditional relativistic magnetron. Numerical and experimental experiments were designed and supported by analytical theory to perform this analysis. Analysis has demonstrated that the 30 ns pulselength of the accelerator at UNM is not sufficient for the low-energy "squeezed state" to form and that a split-cathode driven MDO at UNM operates in a high-energy state. The high-energy state of the electron beam increases the anode lifetime at the cost of reduced output power and efficiency. The downstream reflector eliminated the leakage current and did not suffer from electric breakdown. The split-cathode is flexible and can operate in both high-energy and low-energy states. The absence of the cathode plasma and decreased anode bombardment makes it an ideal candidate for a rep-rated operation.
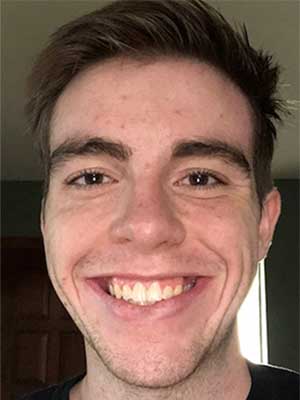
Nathan Patrizi [Spring 2022]
02-23-2022
Biography
Nathan Patrizi defended his Ph.D. Dissertation, "Decentralized Intelligent Decision Makingin Cyber Physical Social Systems" in a Zoom meeting chaired by Dr. Eirini Eleni Tsiropoulou on February 23 at 8:30 am.
Abstract
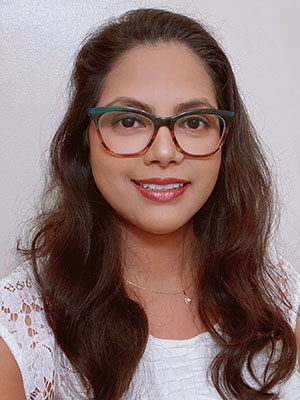
Khandakar Islam [Spring 2022]
02-11-2022
Biography
Khandakar Islam defended her dissertation, "Analytical, Simulation, and Experimental Study of the Generation of Multiple Beams for Application to a Traveling Wave Tube" on Tuesday, February 15 at 10:30 am in a meeting at ECE chaired by Dr. Edl Schamiloglu.Abstract
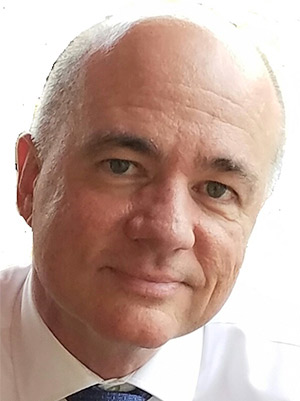
Antonio Gomez [Spring 2022]
12-17-2021
Biography
Antonio Gomez defended his dissertation, "Speaker Diarization and Identification from Single-Channel Classroom Audio Recording Using Virtual Microphones" in a Zoom meeting chaired by Dr. Marios Pattichis on Friday (Dec 17) at 11 am.Abstract
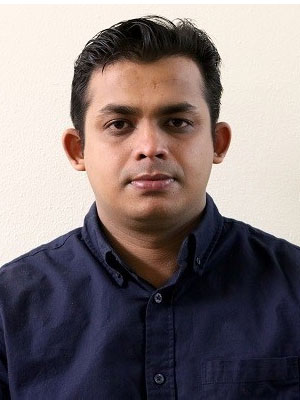
Preyom Dey [Spring 2022]
12-10-2021
Biography
Preyom Dey defended his PhD Dissertation, "Synthetic Aperture Optical Imaging Interferometric Microscopy with Improved Image Quality" in a Zoom meeting chaired by Dr. Steven Brueck on Friday (Dec 10) at 1 pm.Abstract
The resolution limit of optical microscopy can be extended by using Imaging Interferometric Microscopy (IIM), which uses a low numerical aperture (NA) objective lens to achieve resolution equivalent to that of a high-NA objective lens with multiple sub-images. Along with the resolution enhancement challenge, IIM often suffers from poor image quality. In this dissertation, several image quality improvement methods are first proposed and verified with simulation and experiment. Next, techniques to extend the resolution limit of IIM to ≤ 100nm using a low-NA objective lens are demonstrated. An experimental technique of using a grating coupler on a planar waveguide is also demonstrated to extend the resolution limit of IIM. Lastly, the capability of IIM is demonstrated by imaging arbitrary structures in a biological sample where a resolution of ≤ 300nm is achieved. This extended resolution of IIM with high image quality will be helpful in optical metrology and biological research.
Dimitrios Sikeridis [Fall 2021]
11-05-2021
Biography
Dimitrios Sikeridis defended his dissertation, "Intelligent Internet of Things Frameworks for Smart City Safety" on Friday, November 5 at 11 am in Zoom Meeting chaired by Dr. Michael Devetsikiotis.Abstract
The emerging Smart City ecosystem consists of a vast edge network of Internet of Things (IoT) devices that continuously interact with mobile devices carried by its citizens. In this setting, the IoT infrastructure, apart from the main communications facilitator, acts as a crowdsourcing mechanism that collects massive amounts of user data, and can support public safety applications for the Smart City. In this thesis, we design and analyze learning mechanisms that extract intelligence from crowd interactions with the wireless IoT infrastructure, and optimize its energy efficiency while operating as a public safety network. First, we deploy a multi-story facility testbed and perform an extensive real-subject trial to gather user interactions with a realistic IoT infrastructure. The resulted BLEBeacon dataset is a collection of Bluetooth Low Energy (BLE) advertisement packets generated from BLE beacons carried by people following their daily routine. To aid fingerprinting localization services, instead of extensive offline measurements, we use the gathered unlabeled Received Signal Strength Indication (RSSI) samples to design a user localization and mobility tracking framework that relies on unsupervised learning.
Following that, we study the transformation of the Smart City's underlying IoT network (edge devices and user equipment) into a Public Safety Network (PSN) able to provide resilient communications under disaster recovery scenarios. We first propose a heterogeneous device-to-device PSN framework that utilizes reinforcement learning to select the most appropriate wireless protocol, in terms of topology, protocol specifications, and battery-life extension. The framework also utilizes a coalition formation approach that considers social relations among devices, physical distance, and energy availability. Each device’s optimal transmission power is obtained through the formulation of a utility-based power control problem as a non-cooperative, distributed game among IoT devices, that converges to a unique Nash equilibrium. The second PSN framework we propose builds on this power management and combines Unmanned Areal Vehicle (UAV)-support with wireless powered communication (WPC) techniques to further improve energy efficiency. The IoT devices use reinforcement learning to form clusters and actively associate with a cluster-head. Towards extending the PSN’s lifetime, we utilize a harvest-transmit-store WPC mechanism, the UAV optimal positioning in the Euclidean 3D space is determined through an optimization problem of maximizing the coalition head’s total energy availability.
Finally, we propose an extensive form perfect information game to model interactions and optimal city resource allocations when a Terrorist Organization (TO) performs attacks on multiple targets across two conceptual Smart City (SC) levels, a physical, and a cyber-social. The Smart City Defense Game (SCDG) considers two SC agencies that have to defend their physical or social territories respectively, and fight against a common enemy, the TO. Each layer consists of multiple targets and the attack outcome depends on whether the resources allocated there by the associated agency, exceed or not the TO's. Each player's utility is equal to the number of successfully defended targets. The two agencies are allowed to make budget transfers provided that it is beneficial for both. We completely characterize the Sub-game Perfect Nash Equilibrium (SPNE) of the SCDG that consists of strategies for optimal resource exchanges between SC agencies and accounts for the TO's budget allocation across physical and cyber targets.
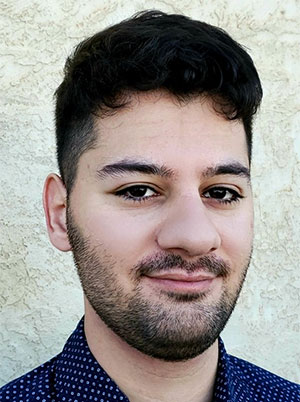
Marios Patriotis [Fall 2021]
08-03-2021
Biography
Marios Patriotis defended his dissertation, "Reconfigurable Antennas & Matching Networks for CubeSats" on Tuesday, August 3 at 9 am in a Zoom Meeting chaired by Dr. Christos Christodoulou.Abstract
Telecommunication has rapidly evolved in the past decades, quickly becoming one of the most expansive industries, especially with the presence of new attractive eras like CubeSats. The communication between a cluster of satellites is a challenging task due to the continuously varying physical, and therefore the electromagnetic environment that they exist in. This issue can be mitigated with the installment of a type of antenna, known as “Reconfigurable Antenna”. These antennas can have agile radiating properties on demand in order to adjust in each new situation.
This dissertation aims to provide a series of reconfigurable antennas able to perform with the diverse radiating properties of frequency, radiation pattern, and polarization, for the CubeSat units enabling them to establish successful communication links among other units but also the grand stations.
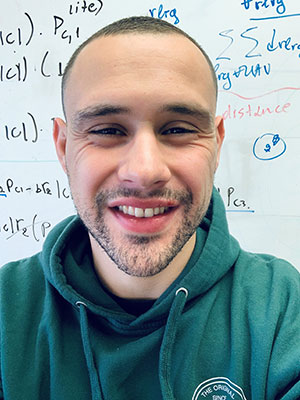
Pavlos Apostolopoulos [Summer 2021]
06-01-2021
Biography
Mr. Pavlos Apostolopoulos defended his PhD Disertation "Artificial Intelligent Risk-aware Autonomous Decision-Making in Resource-Constrained Computing Systems" in a Zoom meeting chaired by Dr. Eirini Eleni Tsiropoulou on Tuesday, June 1 at 9 am.Abstract
Artificial Intelligent autonomous systems are becoming increasingly ubiquitous in daily life. Mobile devices for example provide mechanical-generated intelligent support to humans, with various degrees of autonomy, and are a key part of the recent autonomous revolution. Autonomous intelligent systems aim to understand and interact with their users in a timely manner, while many of them are characterized by constrained resources. Despite that, the average person does not act in a formulaic and risk-neutral manner but instead exhibits risk-aware attitudes when performing a task that includes sources of uncertainties. When humans make decisions, they explore their surroundings, understand the emerging risks, perform actions, and evaluate their perceived outcomes. What a person characterizes as a satisfactory outcome is subjective to her own reasoning, behavior, and risk capacity. Therefore, an autonomous intelligent system should be enriched with human awareness, thus it should account for and sometimes mimic its owner's cognitive behavior and behavioral patterns, such that the latter's subjective satisfaction is optimized, and personalized service is provided.
Furthermore, the proliferation of autonomous systems, e.g., mobile or wearable devices, boosts the data volume and service demand. Each autonomous system aims to optimize its owner's experience in a self-centric manner, and in several application domains, its actions impact the others' experience and decision-making process generally. To this end, the users' subjective goals generate conflicts, and the autonomous intelligent systems are expected to make decisions in non-cooperative environments. In this thesis, we investigate and introduce distributed autonomous decision-making frameworks by focusing on motivating application domains with the aforementioned challenges. We utilize Game Theory for studying the strategic interaction of the autonomous intelligent systems in non-cooperative environments and tackling the necessity of non-centralized and scalable solutions. We build autonomous intelligent decision-making agents through Reinforcement Learning, which is a popular statistical Artificial Intelligence (AI) technique for controlling unknown environments with partial, and incomplete information. Reinforcement Learning (RL) introduces the concept of an agent that learns to interact with an unknown environment by performing actions that are mainly driven by particular observations, and by evaluating the resulted feedback. We extend the regular RL setting through reward reshaping for considering the user's risk-aware characteristics that are exhibited in real life. We incorporate Prospect Theory, which belongs to the behavioral economic subgroup, and describes how individuals make decisions between probabilistic alternatives, where risk is involved, and the probability of different outcomes is unknown. In the considered non-cooperative environments, we seek distributed solutions, thus Equilibrium points, where each autonomous intelligent agent does not have the incentive to change its own decision unilaterally.
Our investigation leads to autonomous intelligent decision-making frameworks that could serve as a step towards Artificial General Intelligence (AGI), where the computing systems learn to perform a task in a human-centric manner, thus in a similar way that the task would be completed by a person in real life.
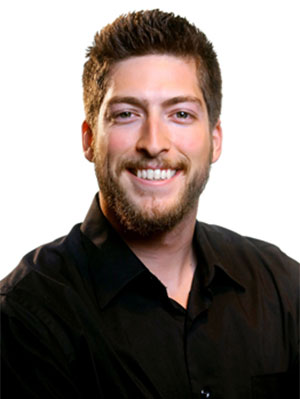
Gabriel Shipley [Spring 2021]
03-01-2021
Biography
Gabriel Shipley defended his PhD dissertation "Timescales to Stabilize and Magnetize Pulsed-Power-Driven Liner Implosions" on Monday (March 1) at 1:30 in a Zoom meeting chaired by Dr. Mark Gilmore.Abstract
This dissertation presents analysis of experiments and simulations executed to develop the auto-magnetizing liner concept (AutoMag) for use as an alternative premagnetization mechanism for MagLIF. Tests of each stage of AutoMag (magnetization, dielectric breakdown, and implosion) were executed on the Mykonos accelerator and the Z accelerator. Experiments demonstrate strong internal axial magnetic field production (20 – 150 T), dielectric breakdown initiation that depends on global induced electric field across the target, and a level of cylindrical implosion uniformity high enough to be useful for prospective fusion-fuel-filled (auto-magnetized MagLIF) experiments.
This dissertation also presents detailed simulations of the Solid Liner Dynamic Screw Pinch concept formulated to mitigate instability growth in liner implosions on Z. 3D MHD simulations were executed to design a feasible target to field in Z experiments. Simulations indicate that instability mitigation is proportional to the cumulative dynamic rotation of the magnetic vector field at the liner surface throughout implosion.
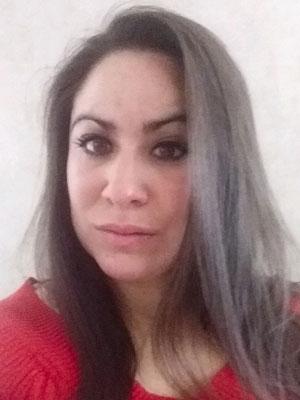
Monica Jaramillo [Spring 2021]
01-15-2021
Biography
Monica Jaramillo defended her dissertation, "Computational Electromagnetics Modeling of Foliage Penetration" in a Zoom meeting that took place on Friday (Jan 15) at 1 pm. Dr. Christos Christodoulou chaired the Committee.Abstract
Foliage penetration (FOPEN) radar at lower frequencies (VHF, UHF) is a well-studied area with many contributions. However, there is growing interest in using higher Ku-band frequencies (12-18 GHz) for FOPEN. Specifically, the reduced wavelength sizes provide some key saliencies for developing more optimized detection solutions. The disadvantage is that exploiting Ku-band for FOPEN is complicated because higher frequencies have pronounced scattering effects due to their smaller wavelengths.
In this dissertation, a methodology is presented for modeling and simulating FOPEN problems in the Ku-band. Unprecedented comprehensive computer-aided design (CAD) tree models were specifically designed to be implemented for characterizing the phenomenology of Ku-band electromagnetic (EM) wave transmissions through moderate foliage. Explicit tree structures are modeled since smaller details have more of an influence at this frequency. Also, moderate foliage is of most interest because with less dense foliage there is a higher percentage of Ku-band transmission through openings in the foliage.
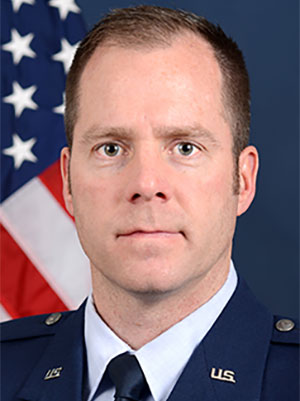
David Hensley [Spring 2021]
04-01-2021
Biography
David Hensley defended his dissertation, "A Reconfigurable, Stretchable Liquid Metal Antenna, Phase Shifter, and Array for Wideband Applications" in a Zoom meeting chaired by Dean Christos Christodoulou on Thursday, April 1 at 1 pm.Abstract
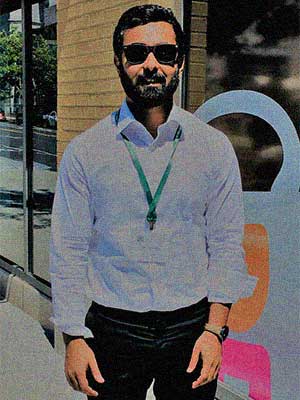
Arjun Gupta [Spring 2021]
01-25-2021
Biography
Arjun Gupta defended his PhD dissertation on Monday, January 25 at 9 am in a Zoom Meeting chaired by Dr. Christos Christodoulou. The title of Mr. Boynton's dissertation is, "Spatial Interpolation and Source Localization with Machine Learning."
Abstract
Source localization using array signal processing has found applications across do mains beginning with radar and sonar to astronomy, acoustics, medical devices, and more recently in autonomous cars and adaptive communication systems. The information about the spatial spectrum can not only provide information about the source and interference but also assists in increasing signal integrity and avoid interference. This provides an added degree of freedom in the form of spatial diversity. This research investigates spatial spectrum estimation of waveforms from the signals sam pled by arbitrarily distributed sensors. Conventional high-resolution algorithms such as root-MuSiC fail to perform accurate source localization due to the reliance of the polynomial rooting technique on the Vandermonde structure of the array manifold. Spatial interpolation methods to estimate the uniform equivalent from the irregularly sampled signal has been widely used to address the irregularity in sampling. We investigate the drawbacks of the current approaches and propose more efficient and robust interpolation methods. Gaussian Process (GP) regression formulation is investigated as an alternative to the existing methodology. The complex variables in communication signals is addressed by introducing a multitask GP formulation instead of using the prevailing complex GP with kernels method. We further explore support vector regression (SVR) and introduce a complex SVR formulation endowed with Mercer's Kernels to perform spatial interpolation. From conventional machine learning we move on to investigate deep learning strategies and propose an encoder decoder architecture for interpolating and denoising. We integrate the interpolation methods with high resolution source localization technique root-MuSiC and develop novel algorithms to perform source localization with arrays of any shape and size. From two step methodologies of spatial interpolation and subsequent angle of arrival estimation we delve into data driven approaches and propose an end-to-end frame work for source localization using a long short term memory (LSTM) architecture. The proposed framework is entirely data driven and can perform seamless source localization with sensor arrays of any given geometry.
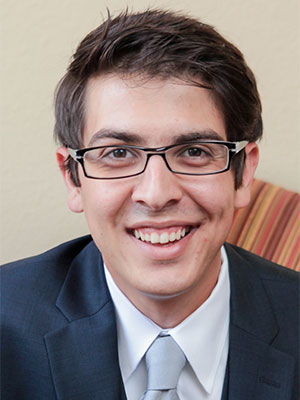
Nicholas Boynton [Spring 2021]
03-29-2021
Biography
Nicholas Boynton defended his PhD dissertation on Monday, March 29 at 1 pm in a Zoom Meeting chaired by Dr. Daniel Feezell. The title of Mr. Boynton's dissertation is, "Heterogeneously Integrated Photonic Modulators and High Volume Manufacturing of Related Technologies."
Abstract
Silicon photonics is an attractive approach to cost-effective integrated optics due to the infrastructure established for silicon CMOS electronics. The material properties of silicon however are not ideal for optical devices. Conversely, lithium niobate is an excellent optical material due to strong Pockels effect but is a notoriously difficult material to process. One method of simultaneously overcoming the material limitations of silicon and the fabrication limitations of lithium niobate is the heterogeneous integration of the two platforms. In this dissertation we review an architecture which integrates thin-film lithium niobate and silicon photonics via bonding to simultaneously address the manufacturing difficulties associated with lithium niobate, and material property limitations associated with silicon.
Variation in the bonding surface is discussed, and mitigation strategies are implemented to improve uniformity. Characterization methods of waveguide-based devices is explored as well in order to identify systematic process variations.
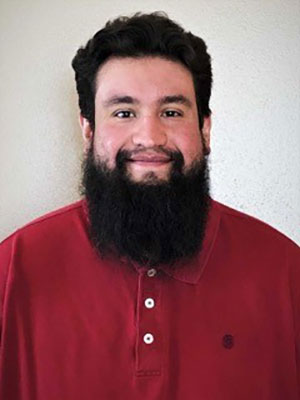
Andrew Aragon [Spring 2021]
04-02-2021
Biography
Andrew Aragon defended his PhD thesis on Friday, April 2 at 9 am in a Zoom Meeting chaired by Dr. Daniel Feezell. The title of Mr. Aragon's dissertation is, "Etched-and-regrown diodes on m-plane GaN for next Generation power electronic devices."
Abstract
Demand for next-generation power electronic devices is driven by continually evolving requirements of power systems. Devices utilizing III-nitride materials (GaN) and vertical selective-area doped architectures are advantageous due to their wide-bandgap, thermal management, small form-factor, and current handling.
Such devices incorporate junctions at multiple crystalline planes. Thus, effects of impurity contamination and etch damage are investigated on the m-plane (10-10) of GaN. Impurites (Si, O, and C) are shown to reduce blocking voltage (~ 102 ×) and increase forward leakage current (~ 104 ×) in regrown versus continuously-grown p-n diodes. Elevated deep level defects at Ec – 1.9, 2.9, and 3.3 eV are identified with increased reverse leakage (~ 103 ×) in etch-and-regrown versus continuously-grown Schottky diodes. Post-dry-etch methods are used to reduce defects and reverse current leakage (~ 10 – 103 ×). Additionally, leakage current mechanisms are investigated via an RF method to extract dynamic parameters of etched-and-regrown p-n diodes.
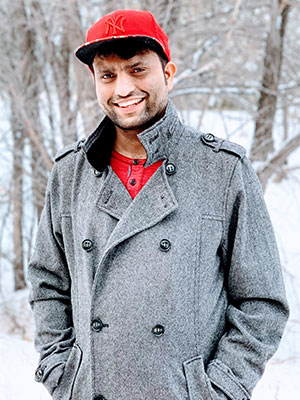
Manish Bhattarai [Fall 2020]
11-05-2020
Biography
Mr. Manish Bhattarai defended his PhD thesis on Thursday, November 5 at 10:30 am in a Zoom meeting chaired by Dr. Manel Martinez-Ramon. The title of Mr. Bhattarai's dissertation was "Integrating deep learning and augmented reality to enhance situational awareness in firefighting environment."
Abstract
Intelligent detection and processing capabilities can improve the safety, efficiency, and successful completion of rescue missions conducted by firefighters in emergency first response settings. This research aims to enhance firefighters’ situational awareness by creating an automated system that combines the latest state-of-the-art deep learning networks and infrared imagery gathered on the scene by firefighters. The newly developed algorithm is capable of real-time, intelligent object detection, recognition, tracking, and segmentation. The processed results are used as inputs in the creation of an augmented reality capable of advising firefighters of their location and key features around them, vital to the rescue operation at hand, as well as a path planning feature that acts as a virtual guide to assist disoriented first responders in getting back to safety.
First, we used a deep Convolutional Neural Network (CNN) system to classify and identify objects of interest from thermal imagery in real-time, which can accurately inform firefighters’ decision-making process with up-to-date scene information by extracting, processing, and analyzing crucial information.
Second, we extended this CNN framework for object detection, tracking, and segmentation with a Mask RCNN framework. Utilizing the new information pro- duced by the framework, firefighters can make more informed inferences about their environment that will improve those decisions that are crucial to their safe naviga- tion through such hazardous and potentially catastrophic environments. We take this system further by combining the object recognition framework with an Natural Language Processing(NLP) model for caption generation to provide the firefighter with descriptions of important scene characteristics gathered by the CNN.
Third, we built a deep Q-learning-based agent, immune to stress-induced disori- entation and anxiety, which was able to make clear navigation decisions based on the observed and stored facts in live-fire environments. As a proof of concept, we imitated structural fire in a gaming engine called Unreal Engine. This virtual setting enabled accelerated learning of the environmental conditions through the agent’s in- teraction with the simulated fire conditions built into the game space. The agent is trained with a deep Q-learning algorithm based on a set of rewards and penalties derived from the gaming agent’s actions on the environment.
Fourth and finally, we use a low computational unsupervised learning technique called tensor decomposition to perform meaningful feature extraction in real-time. This method allows for rapid assessment of anomalous events recorded by the camera that can be processed much more quickly than data interpreted through the CNN. The information gained from this technique can be used as a warning system for firefighters to alert them to rapidly changing conditions such as room temperature or smoke prevalence. The method can be deployed with lower power, memory and computational requirements but achieve similar accuracy. Tensor decomposition aid the recognition process by decomposing the sensor data along each dimension for blind source separation and anomaly detection.
When combined, these four approaches present a new approach to information understanding, transfer, and synthesis that could dramatically improve firefighter response and efficacy and reduce the loss of life.
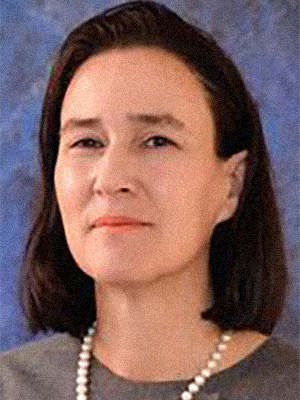
Andrea Schmidt [Fall 2020]
09-13-2020
Biography
Ms. Andrea Schmidt defended his PhD thesis on Friday, November 13 at 9 am in a Zoom meeting chaired by Dr. Edl Schamiloglu. The title of Ms. Schmidt's dissertation was "Theoretical and Experimental Studies of the Emission of Electromagnetic Radiation by Superluminal Polarization Currents."
Abstract
Recent experiments, conducted at Los Alamos National Laboratory, have shown that polarization currents (i.e., ∂P/∂t, where P is formally defined as the dipole moment per unit volume), accelerated to superluminal speeds within a dielectric solid, emit tightly focused packets of electromagnetic radiation. Extended faster-than-light sources are distinct from emitters that employ surface currents of free electrons on localized elements such as dipoles to produce radiation. They are true volume sources whose radiation characteristics are entirely dependent on the speed and acceleration of the moving polarization along with the size, shape, and dielectric constant of the solid that contains it. Prototype general-purpose, active antennas that employ extended distributions of polarization currents moving faster than light have already demonstrated advantages over conventional technologies in communications and radar systems.
After a brief introduction, the present talk will focus on the mathematical treatment of extended faster-than-light sources of electromagnetic radiation, presenting ab-initio calculations and suggesting distributional as well as numerical solutions. This work was motivated by the fact that data collected from our practical emitters did not match the predictions made by others in the field; here we will carefully examine their arguments and rectify spurious claims that were made during the infancy of this new and vibrant field of research.
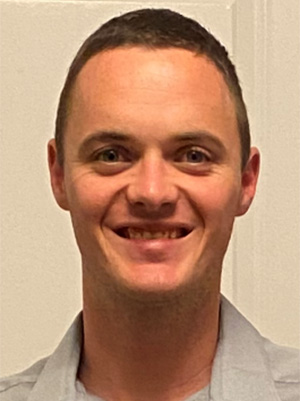
Derek Heeger [Fall 2020]
09-12-2020
Biography
Mr. Derek Heeger defended his PhD thesis on Thursday, November 12 at 2 pm in a Zoom meeting chaired by Dr. Jim Plusquellic. The title of Mr. Heeger's dissertation was "Mesh Networking and Security Applications in LoRa Ad-Hoc Networks."
Abstract
The Internet of Things (IoT) has rapidly expanded into consumer and industratial applications driving the need for secure and energy efficient communications suitable for battery powered devices. Common IoT protocols developed for these applications include SigFox, Narrow-Band IoT, and Long Range (LoRa). LoRa is an energy efficient long-range communication method enabled by advanced modulation schemes. This research enables new capabilities in LoRa ad-hoc networks allowing for innovative IoT sensors that were previously infeasible due to energy constraints. This is achieved by investigating adaptive data rate (ADR) techniques, enabling reliable and secure remote firmware updates, improving LoRa authenication, and implementing a secure mesh network topology suitable for mobile, energy-constrained devices. These concepts are developed, analyzed, and then applied to an advanced cattle monitoring sensor that tracks the health and location of free-range cattle.
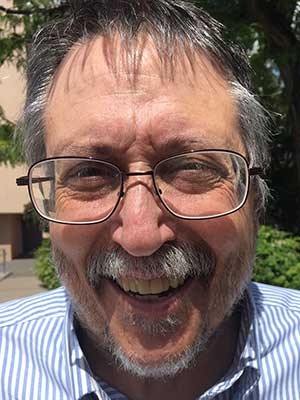
Eric Hamke [Fall 2020]
09-14-2020
Biography
Mr. Eric Hamke defended his PhD thesis on Monday, September 14 at 11 am in a Zoom meeting chaired by Dr. Ramiro Jordan. The title of Mr. Hamke's dissertation was "Detecting Physical Stress Markers in Human Speech Using Deep Learning."
Abstract
The focus of the research has been to monitoring breathing and identify changes in pitch to identify physical task markers in speech.
Using a Restricted Boltzman Machine, firefighters' SCBA regulator valve sounds are classified as open and closed. This process also involves retraining periodically to include past observations since the last processing interval. The classifications are combined into continuous intervals. Observing the length of the intervals and the number of interval-starts represents time spent inhaling and the breathing rates (breaths per minute).
Voice pitch estimation using a multilayer LSTM network in a high noise envi-ronment (-10dB) uses a multilayer LSTM RNN classified speech segments as voiced or unvoiced. The classifications are grouped into continuous intervals. The pitch tracking processes uses these intervals in another multilayer LSTM RRN regression to estimate the pitch values and ensure continuity between observed values.
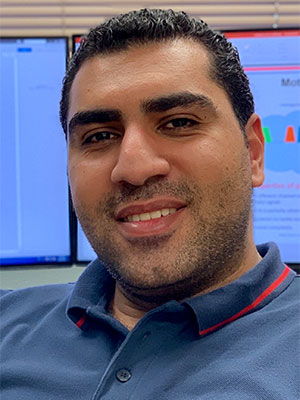
Mohamed Attia Abdelrahman Aref [Fall 2020]
08-07-2020
Biography
Mohamed Attia Abdelrahman Aref defended his PhD thesis on Friday, August 7 at 10 am in a Zoom meeting chaired by Dr. Sudharman Jayaweera. The title of Mr. Aref's dissertation was "Cognitive Radios for Self-aware and Spectrum-agile Communications over Wideband Spectrum."
Abstract
In this dissertation, we introduce a novel cognitive engine design for intelligent, selfaware and spectrum-agile communications. The targeted cognitive radio (CR) has sensing and learning abilities that incorporate advanced signal processing and machine/deep learning (ML/DL) techniques. It can operate over a heterogeneous wideband spectrum that is much wider than the ones proposed in the literature, most likely covering several hundreds of MHz. It has the ability to detect existing signals in the surrounding RF environment and identify their origins. Furthermore, it cognitively uses channel selection, transmit power control and interference cancellation to adapt its communications mode inresponse to observed RF conditions. It can find the best transmission opportunities and maintain a certain level of quality of service (QoS). The proposed cognitive techniques can facilitate the use of intelligent spectrum management where operating licenses are issued based on the radio’s intelligence level. It also can be used in a wide range of applications including RF spectrum awareness, signals intelligence, anti-jamming and interference avoidance.
To this end, we implemented replicated Q-learning sub-band selection method for wideband spectrum based on partially-observable Markov decision process. Simulation results showed that the proposed algorithm can provide a substantial improvement over the random sub-band selection policy with only 16% below the optimal sub-band selection policy that requires complete state observability. A DL classification framework is introduced to distinguish between radar and communications signals based on their cyclostationary features. The proposed approach can reach classification accuracy around 99% at signal-to-noise ratio (SNR) of −4 dB. A multi-agent Q-learning technique is provided for anti-jamming and interference avoidance communications. An optimal energy detector is used based on the Neyman-Pearson (NP) criterion which maximizes the detection probability of existing signals subject to a certain false alarm level. Compared with existing schemes, the proposed approach uses two parallel communications operations: sensing and transmission, that can enable it to predict the locations of the jamming/interference signals accurately and hence avoid them efficiently.
A multi-task transfer deep reinforcement learning (DRL) approach is developed to provide spectrum-agile communications over wideband spectrum where communications over each sub-band represents a single task. The proposed approach attempts to learn an optimal policy for channel and transmit power level selection. The proposed approach has proved that it is suitable for real-time applications with its ability to adapt to sudden changes in the spectrum of interest. It outperforms existing schemes found in literature such as single-task DRL and Q-learning. A DL-aided successive interference cancellation (SIC) framework is proposed for massive multiple-input-multiple-output nonorthogonal multiple access (massive MIMO-NOMA) systems. A better realistic scenario, compared with similar approaches in the literature, that includes correlated channel fading and user mobility based on random waypoint (RWP) model is considered. Simulation results showed that the proposed approach provides a significant reduction in error propagation and computational complexity that might be encountered in traditional SIC schemes including those based on DL.
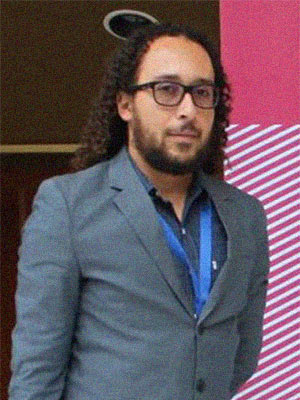
Luis Valbuena Reyes [Fall 2020]
07-15-2020
Biography
Mr. Luis Valbuena Reyes defended his PhD thesis on Wednesday, July 15 at 2 pm in a Zoom meeting chaired by Dr. Edl Schamiloglu. The title of Mr. Reyes' dissertation was "Software Execution under Extreme Electromagnetic Interference."
Abstract
Software routines that deviate from the original algorithm goal due to a perturbation agent is a phenomenon that has been observed for a long time now. From unintended executions on flight control computers during the dawn of space exploration, passing through hardware Trojans tampering in corporate security, to sensitive medical equipment compromised to the point of changing the amount of delivered drug, the time intervals, and the reported biomedical measured data.
In this dissertation, a computer under perturbation is modeled by a hybrid system that captures the digital nature on the discrete part, and the interaction of the perturbation signal with the hardware on the continuous part, like extreme electro-magnetic interference (EEMI) on logic circuits. From this point, the dissertation develops in three directions. First, we develop a testbed simulation environment of a 4-bit processor with the ability to take perturbation signals both in time and space. Second, the formulation of mathematical models of the computer logic that take perturbation signals as inputs; and third, the use of reachability theory to access how far the perturbation propagates through hardware to cause an exploitable deviation in software execution. We formulate two modifications to the traditional Hamilton-Jacobi-Evans equations (HJE). A diffusion term is added to the original HJE and we also include the second-order terms of the Taylor expansion of the optimality principle.
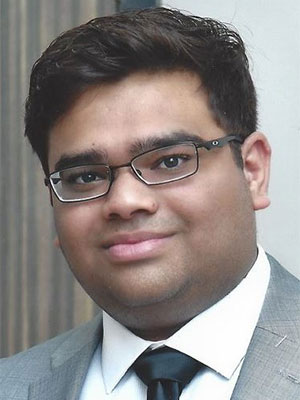
Nishchay Sule [Summer 2020]
06-30-2020
Biography
Nishchay Sule defended his PhD thesis on Tuesday, June 30 at 1 pm in a Zoom meeting chaired by Dr. Payman Zarkesh-Ha. The title of Mr. Sule's dissertation was "Device-level Predictive Modeling of Extreme Electromagnetic Interference."
Abstract
Radio Frequency (RF) interference is a prominent issue for modern electronic devices. As device size and supply power shrink to meet the on-going demand for compact and complex Integrated Circuits (ICs), their susceptibility to external noise coupling to the input or power supply increases significantly. One such type of noise that acts upon a system to be considered is Extreme Electromagnetic Interference (EEMI). Previous works done to understand and evaluate the impact of EEMI onto a system or sub-system have been conducted on a statistical or empirical analysis level, which has led to complex and convoluted analysis, that requires significant time and computational power. Furthermore, since Electromagnetic Interference/Compatibility (EMI/EMC), engineers have to deal with complex systems, they typically come up with an estimate to analyze such systems. The premise behind this research is to highlight the development of the refinements of such "rule-of-thumb" guidelines to help EMI/EMC engineers quickly estimate device or circuit level susceptibility for the injected EEMI signals. A novel analytical model is proposed in this research, which offers an alternative solution for the limits of malfunction for a Silicon-based (Si) Metal-Oxide-Semiconductor Field-Effect Transistor (MOSFET) under EEMI bias.
These developed analytical predictive models help determine the maximum limits for large-signal gate-side (input) or drain-side (power supply) injection in terms of the device's ION/IOFF ratio prior to degradation or damage to the device. The ION/IOFF ratio is a function based on the MOSFETs' device parameters. These models have been developed for a single transistor, particularly n-type and p-type MOSFETs when the EEMI signal is superimposed onto the gate or drain terminals. Additionally, these predictive models have also been extended to determine the maximum tolerance for gate or drain injections as transistor technology scales down. Furthermore, these models have been compared and validated with prototype test chips across five different technology nodes.
Lastly, the analytical models have been expanded to be used in several different assessments, such as high-frequency analysis, manufacturer, and transistor size-independent and sensitivity modeling. Such demonstrations show the fundamental nature and flexibility, which allows these models to be used based on the EMI/EMC engineers' needs.
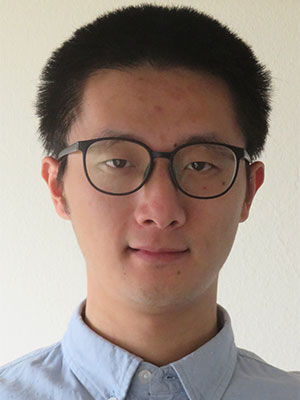
Shu Wang [Summer 2020]
06-22-2020
Biography
Shu Wang defended his PhD thesis on Monday, June 22 at 1:30 pm in a Zoom meeting chaired by Dr. Christos Christodoulou. The title of Mr. Wang's dissertation was "Advanced Parallel Algorithms in Computational Electromagnetics."
Abstract
The rapid development of high performance computing has pushed the computational electromagnetics (CEM) towards high accuracy, high fidelity and extreme computational scales. There is a great need for existing CEM solvers to have enhanced parallelism and scaling capability. The purpose of this dissertation is to investigate advanced parallel algorithms for both frequency and time domain solvers.
In frequency domain, this work first develop the underpinnings of parallel preconditioning technique and high-order transmission condition in the context of multiÂsolver scheme. The result is a computing resource-aware and implementation wise compact solver. Then this work targeted at developing efficient algorithms for cases where iteration of simulations,e.g. parameter sweep, is necessary. The proposed platform Green's function method can effectively reduce the turnaround time by exploiting reusable matrices.
In time domain, due to the ever-increasing sophistication in EM systems, a typical transient simulation may require many time steps. Most current transient solvers exploit parallelism in spatial domain which it is not trivial to sustain parallel scaling capability in high level. This work,therefore, provided a new perspective in parallelism, parallel-in-time(PIT). The problem is first decomposed based on superposition principle and corresponding effective integration methods are developed. Next, a hyÂbrid parallel scheme, space-time building block method, which is based on reduced order model, is proposed for applications like meta-material simulation. A improved scaling efficiency and 3x speed-up is observed in our work. Finally, PIT is extended to improve scaling efficiency for nonlinear circuit-electromagnetic co-simulations, where 2x better efficiency is achieved by proposed algorithms.
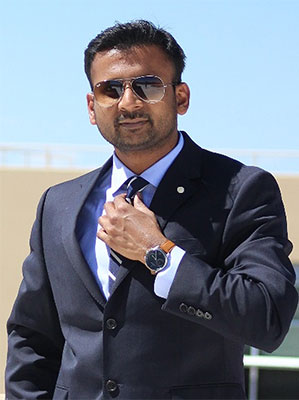
Gangadharan Esakki [Summer 2020]
05-25-2020
Biography
Gangadharan Esakki defended his PhD thesis on Monday, May 25 at 9 am in a Zoom meeting chaired by Dr. Marios Pattichis. The title of Mr. Esakki's dissertation was "Adaptive Encoding for Constrained Video Delivery in HEVC, VP9, AV1 and VVC Compression Standards and Adaptation to Video Content."
Abstract
The dissertation proposes the use of a multi-objective optimization framework for designing and selecting among enhanced GOP conï¬gurations in video compression standards. The proposed methods achieve ï¬ne optimization over a set of general modes that include: (i) maximum video quality, (ii) minimum bitrate, (iii) maximum encoding rate (previously minimum encoding time mode) (iv) and can be shown to improve upon the YouTube/Netflix default encoder mode settings over a set of opposing constraints to guarantee satisfactory performance. The dissertation describes the implementation of a codec-agnostic approach using different video coding standards (x265, VP9, AV1) on a wide range of videos derived from different video datasets. The results demonstrate that the optimal encoding parameters obtained from the Pareto front space can provide signiï¬cant bandwidth savings without sacriï¬cing video quality. This is achieved by the use of effective regression models that allow for the selection of video encoding settings that are jointly optimal in the encoding time, bitrate, and video quality space. The dissertation applies the proposed methods to x265, VP9, AV1 and using new GOP conï¬gurations in x265, delivering over 40% of the optimal encodings in two standard reference videos. Then, the proposed encoding method is extended to use video content to determine constraints on video quality during real-time encoding. The content-based approach is demonstrated on identifying camera motions like panning, stationary and zooming in the video. Overall, the content-based approach gave bitrate savings of 35 % on the zooming & panning motion from Shields video, and 51.5 % on stationary & panning motion from Parkrun video. Additionally, the dissertation develops a segment-based encoding approach that delivers bitrate savings over YouTube’s recommended bitrates. Using BD-PSNR and BD-VMAF, a comparison is made of x265, VP9, AV1 against the emerging VVC encoding standard. The new VVC-VTM encoder is found to outperform all rival video codecs. Based on subjective video quality assessment study, AV1 was found to provide higher quality than x265 and VP9.
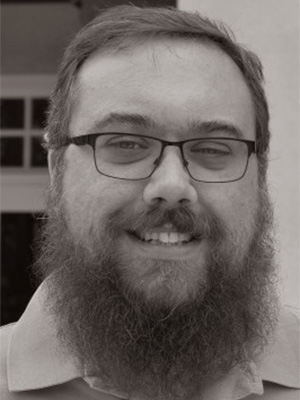
Jon Cameron Pouncey [Spring 2020]
03-27-2020
Biography
Jon Cameron Pouncey defended his PhD thesis on Friday, March 27 at 10 am in room 118 of the ECE Building. Dr. Jane Lehr served as his committee chair. The title of Mr.Pouncey's dissertation is, "Enabling Compact Pulsed Power."
Abstract
The first two decades of the 21st century have seen significant interest in expanding the application of pulsed power technology beyond traditional uses in physics and nuclear weapons research. Applications in the field of national defense, which present unique constraints on system size, have provided impetus to increase the exploration of compact pulsed power solutions. Innovations related to energy density, insulation, switching, and power conversion systems have been realized, bringing deployable compact pulsed power systems closer to realization than ever before. However, recent systems integration efforts have shown that research into tools and technologies is still needed to unlock the full potential of compact pulsed power. This thesis describes efforts to make contributions to three of these research needs.
The first need is for tools that improve predictive simulation of compact pulsed power systems. The principal challenge in satisfying this need is to develop simulation models of components unique to pulsed power systems for use in well-known simulation tools. In order to address this challenge, a predictive parametric model of a spark gap switch has been developed and validated for use in the ubiquitous SPICE circuit simulation software.
The second need is to formalize a set of design principles for compact pulsed power systems. The principal challenge in satisfying this need is to improve understanding of the behavior of pulsed power systems as they are made significantly more compact. This understanding can then be applied early in the development of systems to improve confidence that the system will not require significant redesign late in the development cycle. In order to address this challenge, a detailed analysis of the erection behavior of the compact Marx generator was completed, and translated into design improvement recommendations.
The third need is to leverage new technologies developed for commercial applications to advance compact pulsed power. The principal challenge in satisfying this need is to identify and assess technologies for applicability to compact pulsed power systems. In order to begin addressing this challenge, a series of novel experiments using a newly developed commercial infrared diode-pumped solid-state micro-laser to trigger gas switches were conducted.
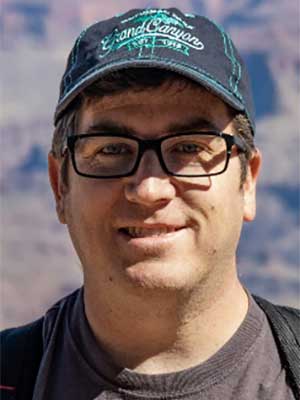
Mitchell Martin [Spring 2020]
04-08-2020
Biography
Mitchell Martin defended his PhD thesis on Wednesday, April 8 at 10 am in a Zoom video conference. Dr. James Plusquellic served as her committee chair. The title of Mr. Martin's dissertation is, "Physical Unclonable Functions Based on Delay Paths and an Interdigital Microstrip Notch Filter."
Abstract
Physical Unclonable Functions Based on Delay Paths and an Interdigital Microstrip Notch Filter
Abstract: A physical unclonable function (PUF) is an integrated circuit hardware primitive that is designed to leverage naturally occurring variations to produce a random bitstring. The arbiter (ARB) PUF is one of the first to be described in the literature. It derives its entropy from variations that occur in the delays of identically configured logic paths. The ARB PUF uses a phase comparator to decide which path of a pair is faster under a given challenge and generates a 0 or 1 as a response indicator bit. Unfortunately, the ARB PUF is not reliable, requiring error correction in cases where the sequence of response bits (the bitstring) needs to be reproduced. In this proposal, a test structure is described, called a time-to-digital converter (TDC) that can measure the actual delays of the paths. This type of ’soft’ information can be used to improve the reliability of the ARB PUF. Data obtained from a set of chips fabricated in IBM’s 90 nm technology, and collected across 9 temperature-voltage corners, is used to demonstrate its effectiveness.
Current PUF designs are typically implemented in silicon like the ARB PUF or utilize variations found in commercial off-the-shelf (COTS) parts. Because of this, existing designs are insufficient for the authentication of Printed Circuit Boards (PCBs). In this thesis, we also propose a novel PUF design that leverages board variations in a manufactured PCB to generate unique and stable IDs for each PCB. In particular, a single copper trace is used as a source of randomness for bitstring generation. The trace connects three notch filter structures in series, each of which is designed to reject specific but separate frequencies. The bitstrings generated with both the ARB PUF and the PCB PUF are evaluated using statistical tests that measure randomness, uniqueness, and reliability.
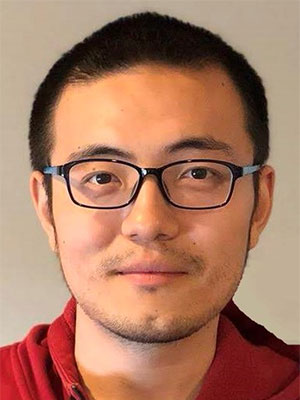
Ran Luo [Spring 2020]
03-16-2020
Biography
Ran Luo defended his PhD thesis on Monday, March 16 at 12:30 pm in room 118 of the ECE building. Dr. Yin Yang served as his committee chair. The title of Mr. Luo's dissertation is, "Advancing Elastic Solid Dynamics in Computer Graphics."
Abstract
This dissertation proposes novel algorithms and applications and provides a real-time and easy-to-use simulator for realistic animation of the 3D solid model. The Finite Element Method (FEM) is a popular tool in the community because of its accurate result, however, the FEM is computationally expensive to handle a large number of DOFs. We present novel techniques to combine linear and nonlinear elasticity with model reduction to provide fast and realistic animation. On the other hand, one of the most important computation tasks of solid simulation is to evaluate the gradient vector and Hessian matrix of elastic energy function. We present a numerical routine to simplify the implementation of solid simulation with the complex-step finite difference (CSFD) that avoids subtractive cancellation. The complexity of nonlinearity is also an obstacle, and we provide a framework called NNWarp to combine the linear elasticity and neural network-based warping method to avoid expensive nonlinear optimization. We also propose an acoustic-VR system as the application. The system can convert acoustic signals of human language to realistic 3D tongue animation in real-time. The Deep Neural Networks (DNN) helps to convert the input speaking voice to positions of pre-defined EMA sensors. Then, a novel reduced physics-based solid simulator, introduced in previous, is used to synthesis the tongue animation.
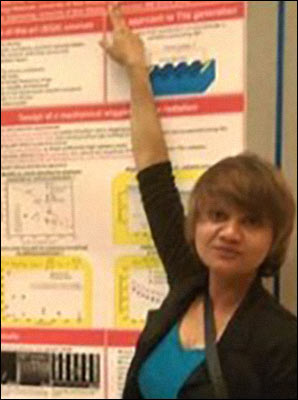
Farhana Anwar [Spring 2020]
03-10-2020
Biography
Farhana Anwar defended her PhD thesis on Tuesday, March 10 at 1 pm in the CHTM building. Dr. Ashwani Sharma served as her committee chair. The title of Ms. Anwar's dissertation is, "Tunneling and Transport Properties of Graphene."
Abstract
This article provides a new method for computing electronic transport properties of graphene i.e. the peculiar tunneling properties of two-dimensional massless Dirac electrons. We consider a simple situation: a massless Dirac electron incident on a potential barrier which is tilted by applied bias and use finite difference method to obtain transmission probability(without involving transfer matrix). In the presence of an applied bias transmission coefficient and tunneling current were obtained and the effect of electric field which modulates the barrier profile therefore conductivity pattern were explained. Furthermore, this method can also be applied to investigate transport properties of disordered graphene as well as device characteristics of room temperature ballistic graphene field-effect transistors. Our study opens up a possibility for graphene-based device optimization by engineering barrier (gate) geometry for graphene MOSFETs as well as devices exploiting optics like behavior of Dirac fermions. In this work we show that finite difference method can be used as an effective approach in low dimensional semiconductor physics.

Joseph Gleason [Fall 2019]
11-06-2019
Biography
Joseph Gleason defended his PhD thesis on Wednesday, November 6 at 4:30 pm in room 118 of the ECE building. Dr. Meeko Oishi served as his committee chair. The title of Mr. Gleason's dissertation is, "Software Design for Probabilistic Safety: Stochastic Reachability and Circadian Control."
Abstract
Reachability is an important verification tool for that provides guarantees of safety while driving the system toward a target. For stochastic systems, in which there is some stochastic disturbance present in the dynamics, we seek controllers that provide a maximal likelihood of safety. Stochastic reachability analysis seeks to a) solves for controllers that maximize the likelihood that a system will reach a target state while staying in safe set, or b) determine the set of initial states for which there exists a controller that can reach a target while staying safe with at least a certain likelihood. Many domains are interested in solutions to stochastic reachability problems. An important example are biomedical systems. Because these systems interact with humans or are models that represent human biological functions, safety in control design is paramount. For biomedical systems, assurances of safety is a difficult because many dynamical models of biological systems are often not well known and the health impacts of violations to safety constraints can have catastrophic consequences. Reachability analysis can provide a methodological framework that can work as the engineering equivalent of "do no harm".
This thesis covers topics in reachability and analysis of human circadian rhythms,i.e. the human sleep-wake cycle. We combine theoretical development with software implementation for both domains.
For stochastic reachability we propose methods for approximating stochastic reachable sets using Lagrangian, set-based, methods. These allow for very fast computation in low-dimensional systems when using polytopic sets, but provide conservative results. These methods utilize recursions that only use basic set operations. The theoretical development is applicable to nonlinear systems, but implementation is restricted to linear systems. We also describe SReachTools, a stochastic reachability toolbox which includes these Lagrangian methods as well as many other approximate and exact solutions for stochastic reachability problems.
In the biomedical domain, we examine methods for determining circadian phase in traumatically brain injured subjects and describe the implementation of a testbed designed to facilitate experimentation in circadian control. For assessing circadian rhythms we use continuously gathered biological signals to allow for a continuous assessment of circadian phase. We additionally demonstrate a unique correlation between circadian power and subject outcome. The lighting testbed details many design considerations for implementing a circadian control facility and demonstrates its ability to operate and its efficacy on phase advancing subjects with a simple pilot study. Both of these work toward an ultimate goal of being able to integrate assurances of safety when using feedback-based lighting to regulate human circadian cycles. This is an area of ongoing and future work.
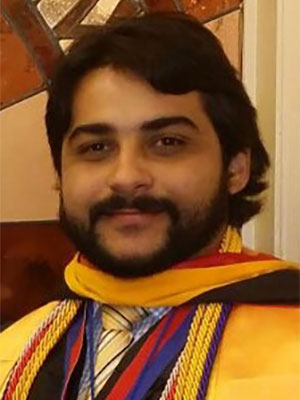
Juan J. Faria-Briceno [Fall 2019]
11-04-2019
Biography
Juan J. Faria-Briceno defended his PhD thesis on Monday, November 4 at 9:30 am in room 101 of the CHTM building. Dr. Steven Brueck served as his committee chair. The title of Mr. Faria-Briceno's dissertation is, "Optical Angular Scatterometry: In-line Approach for Roll-2-roll and Nano-imprint Fabrication Systems."
Abstract
As critical dimensions continue to shrink and structures become more complex, metrology processes are challenged to be implemented during in-line nanomanufacturing. Non-destructive, non-contact, and high-speed conditions are required to achieve proper metrology processes during in-line manufacturing. Optical scatterometry is a nanoscale metrology tool widely used in manufacturing. However, most applications of optical scatterometry operate off-line. A high-speed, in-line, non-contact, non-destructive scatterometry angular system has been demonstrated to scan pattern surfaces during real-time nano-fabrication.
Our system has demonstrated scanning capabilities using flat, 1D and 2D structures. The flat surface samples consist (commercially and grown) of thin film native oxide, grown oxide, and alumina. The 1D samples were made by using interferometric lithography with a thin deposited layer (~85 nm) of aluminum. The 2D complex samples are hollow silicon tubes fabricated using nano-imprint lithography. The inside diameter, outside diameter, and the period of the hollow pillars are respectively ~100 nm, ~135 nm and ~200 nm. These are test structures to establish the metrology capabilities. The applicability of the tool is not restricted to these samples.
Our current in-line scatterometer uses 45° off-axis parabolic mirrors which allows us to have an angular range up to ~50°. The system uses a high-speed scanner at 8 kHz to vary the angle of incident of the beam at the focal spot on the moving web. The system uses a 405 nm collimated diode laser. Our scan period is 125 µs which will allow us to scan 20-30 reflectance measurements before the web moves a distance comparable to the focal spot. A biased silicon detector is used to collect the high-speed reflection signal. The data collected is averaged with a digital scope and further processed on the computer for analysis. Our current system can be integrated with nano-imprint and other R2R real-time fabrication techniques. The goal is to improve quality control and monitor real-time high-speed nano fabrication processes. The angular range can be improved (up to 79°) by varying the focal length and the curvature of the parabolic mirrors. The scanning time can be reduced by increasing the frequency of the resonant scanner. Evidently, in-line angular scatterometry offers solutions to the future of R2R semiconductor nanomanufacturing.
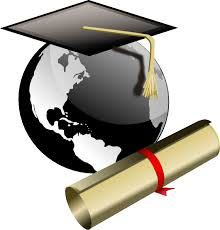
Michael Darling [Fall 2019]
11-01-2019
Biography
Michael Darling defended his PhD thesis on Friday, November 1 at 11 am in room 310 of the ECE building. Dr. Don Hush served as his committee chair. The title of Mr. Darling's dissertation is, "Using Uncertainty To Interpret Supervised Machine Learning Predictions."
Abstract
When a machine learning model generates a prediction, a decision maker needs to determine its validity. Currently, such judgments depend heavily on expert opinion, using a mix of domain and modeling expertise, and accuracy-based validation
metrics. While these methods evaluate a model’s performance relative to a set of validation data, they do not tell us the model’s certainty with respect to a particular prediction on an unseen, perhaps critical, data point.
In this dissertation we develop an uncertainty measure we call minimum prediction deviation which we use to assess the quality of the individual predictions made by supervised two-class classifiers. We show how minimum prediction deviation can be used to di↵erentiate between the samples that a model predicts credibly, and the samples on which an alternate analysis may be required.
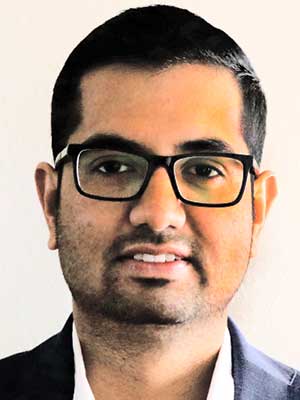
Vijay Saradhi Mangu [Fall 2019]
07-25-2019
Biography
Vijay Saradhi Mangu defended his PhD thesis on Thursday, July 25 at 9 am in room 101 of the CHTM building. Dr. Francesca Cavallo served as his committee chair. The title of Mr. Mangu's dissertation is, "Pixelated GaSb Membranes for Photovoltaics: Fabrication and Structure-Property Relationships."
Abstract
The purpose of this thesis is to develop a reliable and efficient approach to heterogeneous integration of single-crystalline GaSb semiconductors with highly mismatched materials. The mismatch may refer to the crystalline structure and the thermal expansion coefficient of single-crystalline GaSb with respect to the other materials of interest. The strategy of hetero-integration relies on epitaxial lift-off (ELO). My approach prevents formation of extended structural defects that are detrimental to the performance of opto-electronic devices and preserves GaSb growth substrates for potential reuse.
Within my research work, I have overcome some outstanding challenges of epitaxial lift-off of lattice-matched GaSb epitaxial layers through pixelated approach and demonstrated the operation of single-crystalline GaSb photovoltaic devices with a unique architecture on single-crystalline Si substrates.
By leveraging release and transfer of GaSb membranes on Si, I have demonstrated operation of thin-film photovoltaic devices with areas of the of the order of 100sx100s mm2 (i.e., pixelated solar cells). The photo-conversion efficiency of ~ 340x340 mm2 pixelated devices amount to ~2.5%, i.e., a comparable efficiency to a 5 x 5 mm2 homo-epitaxial GaSb cell on a GaSb substrate.
A detailed structure-property relationships study is also performed to justify device characteristics of pixelated GaSb solar cells.
In conclusion, I have established a reliable and efficient process to isolate GaSb epilayers without the formation of any extended defects. I have demonstrated thin films and pixelated GaSb photovoltaic devices on single-crystalline Si substrates and performed a detailed structure-property relationship study to justify their properties and provide a path to improve their performance.
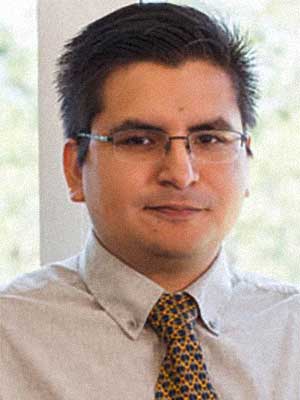
Alvaro Ulloa Cerna [Summer 2019]
07-03-2019
Biography
Alvaro Ulloa Cerna defended his PhD thesis on Wednesday, July 3 at 8 am in room 118 of the ECE building. Dr. Marios Pattichis served as his committee chair. The title of Mr. Cerna's dissertation is, "Large Scale Electronic Health Record Data and Echocardiography Video Analysis for Mortality Risk Prediction."
Abstract
Electronic health records contain the clinical history of patients. The enormous potential for discovery in such a rich dataset is hampered by their complexity. We hypothesize that machine learning models trained on EHR data can predict future clinical events significantly better than current models. We analyze an EHR database of 594,862 Echocardiography studies from 272,280 unique patients with both unsupervised and supervised machine learning techniques.
In the unsupervised approach, we first develop a simulation framework to evaluate a family of different clustering pipelines. We apply the optimized approach to 41,645 patients with heart failure without providing any survival information to the underlying clustering approach. The model separates patients with significantly different survival characteristics. For example, in a 10-cluster model, the minimum and maximum risk clusters had a median survival of 22 and 53 months respectively.
In the supervised approach, with 723,754 videos available from 27,028 unique patients, we assess the predictive capacity of Echocardiography video data for one- year mortality. Also, we hold out a balanced dataset of 600 patients to compare the model performance against cardiologists. We found that the best model, among four candidate architectures, is a 3D dyadic CNN model with an average AUC of 0.78 for a single parasternal long axis view. The model yields an accuracy of 75% (AUC of 0.8) on the held-out dataset while the cardiologists achieve 56% and 61%. The model performance was significantly higher than that of the cardiologists.
Finally, we develop a multi-modal supervised approach that enables interpretability. The model provides interpretations through polynomial transformations that describe the individual feature contribution and weights the transformed features to determine their importance. We validate our proposed approach using 31,278 videos from 26,793 patients. We test our proposed approach against logistic regression and non-linear and non-interpretable models based on Random Forests and XGBoost. Our results show that the proposed neural network architecture always outperforms logistic regression models while its performance approximates the other non-linear models. Overall, our multi-modal classifier based on 3D dyadic CNN and the interpretable neural network outperforms all other classifiers (AUC=0.83).
Saadat M. Mishkat-Ul-Masabih [Summer 2019]
04-24-2019
Biography
Saadat M. Mishkat-Ul-Masabih defended his PhD thesis on Wed, April 24 at 10 am in room 101 of the CHTM Building. Dr. Daniel Feezell served as a committee chair. The title of Mr Saadat M. Mishkat-Ul-Masabih's dissertation is, "Nonpolar GaN-Based VCSELs with Lattice-Matched Nanoporous Distributed Bragg Reflector Mirrors."Abstract
Wide-bandgap optoelectronic devices have undergone significant advancements with the advent of commercial light emitting diodes and edge-emitting lasers in the violet-blue spectral region. They are now ubiquitous in several lighting, communication, data storage, display, and sensing applications. Among the III-nitride emitters, vertical-cavity surface-emitting lasers (VCSELs) have attracted significant attention in recent years due to their inherent advantages over edge-emitting lasers. The small active volume enables single-mode operation with low threshold currents and high modulation bandwidths. Their surface-normal device geometry is conducive to the cost-effective formation of high-density 2D arrays while simplifying on-chip wafer testing. Furthermore, the low beam divergence and circular beam profiles in VCSELs allow efficient fiber coupling.Nevertheless, GaN-based VCSELs are still in the early stages of development. Several challenges need to be addressed before high-performance devices can be commercially realized. One such challenge is the lack of high-quality distributed Bragg reflector (DBR) mirrors. Conventionally, epitaxial and dielectric DBRs are used which often involve complex growth and fabrication techniques. This dissertation provides an alternative approach where subwavelength air-voids (nanopores) are introduced in alternating layers of doped/undoped GaN to form the DBR structure. Selective electrochemical etching creates nanopores in the doped layers, reducing the effective refractive index relative to the surrounding undoped GaN. Using only 16-pairs, DBR reflectance >99.9% could be achieved. Several research groups have shown optically pumped VCSELs using nanoporous DBRs on c-plane. However, there are no reports of electrically injected nanoporous VCSELs. Using m-plane GaN substrates, we have demonstrated the first ever electrically injected GaN-based VCSEL using a lattice-matched nanoporous DBR. The nonpolar m-plane orientation is beneficial for leveraging the higher per-pass gain and polarization-pinning properties absent in c-plane. Lasing under pulsed operation at room temperature was observed at 409 nm with a linewidth of ~0.6 nm and a maximum output power of ~1.5 mW. This is the highest output power from m-plane VCSELs to date with relatively stable operation at elevated temperatures. All tested devices were linearly polarization-pinned in the a-direction with high polarization ratios >0.9. Overall, the nanoporous DBRs help in mitigating some of the issues that limit the performance of III-nitride VCSELs.

Brock Roberts [Spring 2019]
04-15-2019
Biography
Brock Roberts defended his PhD thesis on Monday, April 15, 2019 at 1 pm in room 237 of the ECE Building. Dr. Edl Schamiloglu served as his committee chair. The title of Mr. Robert's dissertation is, "Noise and Gain Characterization of Interband Cascade Infrared Photodetectors."
Abstract
A cavity designed to have multiple harmonic TM0N0 modes can be used to accurately measure the longitudinal profile of a bunched charged particle beam passing through its bore, non-invasively, and in real time.
Multi-harmonic TM0N0 cavities were designed, constructed, and beamline tested in a variety of experiments at the Thomas Jefferson National Accelerator Facility (TJNAF or Jlab). Measurements with a sampling oscilloscope provided signals that resemble the profile of electron bunches passing through the cavity’s bore. Straightforward signal processing techniques reduce distortion in the measurement and provide real time profiles of electron bunches with picosecond accuracy. Subharmonic beams having bunch repetition rates of 1/3rd and 1/6th of Jlab’s 1497 MHz bunch frequency, and interleaved sub-harmonic beams were also measured. Comparison between measurements made using a harmonic cavity were corroborated with an established invasive measurement method and with computer models. A harmonic cavity from this effort has been installed within the CEBAF injector, allowing accelerator operators to view, in real time, the shape and duration of electron bunches entering the accelerator. Another harmonic cavity has been installed within Jlab’s Upgraded Injector Test Facility (UITF), and two more are planned for installation there. This effort was awarded the 2016 International Beam Instrumentation Conference’s Faraday Cup Award.

Francisco German Perez Venegas [Spring 2019]
04-12-2019
Biography
Francisco German Perez Venegas defended his PhD thesis on Friday, April 12 at 2:30 pm in room 118 of the ECE Building. Dr. Balu Santhanam served as his committee chair. The title of Mr. Venegas' dissertation is, "Detection and classification of vibrating objects in SAR images."
Abstract
The vibratory response of buildings and machines contains key information that can be exploited to infer their operating conditions and to diagnose failures. Furthermore, since vibration signatures observed from the exterior surfaces of structures are intrinsically linked to the type of machinery operating inside of them, the ability to monitor vibrations remotely can enable the detection and identification of concealed machinery.
This dissertation focuses on developing novel detection schemes for performing detection and classification of vibrating objects in SAR images. Three are the central claims of this dissertation. First, the non-linear transformation that the microdoppler return of a vibrating object suffers through SAR sensing does not destroy its information. Second, the instantaneous frequency (IF) of the SAR signal has sufficient information to characterize vibrating objects. Third, it is possible to develop a detection model that encompasses multiple scenarios including both monocomponent and multi-component vibrating objects immersed in noise and clutter.
For answering these claims, two different schemes are studied for both the detection and classification of vibrating objects in SAR images. The first scheme is data-driven and utilizes features extracted with the discrete fractional Fourier transform (DFRF T) to feed machine-learning algorithms (MLAs). Specifically, the DFRFT is applied to the IF of the slow-time SAR data, which is reconstructed using techniques of time-frequency analysis. The second scheme is model-based and employs a probabilistic model of the SAR slow-time signal, the Karhunen-Loeve transform (KLT), and a likelihood-based decision function. The performance of the proposed schemes is characterized using simulated data as well as real SAR data collected with the Lynx SAR. The suitability of SAR for sensing vibrations is demonstrated by showing that the separability of different classes of vibrating objects is preserved even after non-linear SAR processing.
Finally, the proposed algorithms are studied in the presence of signal noise and terrain clutter. The results show that the proposed schemes produce high-precision classifiers capable of dealing with noise and clutter of moderate intensity. In order to loosen these requirements, the Hankel rank reduction (HRR) method, previously used for suppressing ocean clutter in ground-wave radar, is adapted to suppress clutter-noise in SAR images.
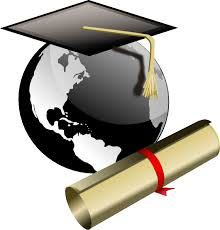
Joshua J. Trujillo, Sr. [Fall 2019]
04-10-2019
Biography
Joshua J. Trujillo, Sr. defended his PhD thesis on Wednesday, April 10 at 11:30 am in room 118 of the ECE building. Dr. Payman Zarkesh-Ha served as his committee chair. The title of Mr. Trujillo's dissertation is, "Study of Distributed Versus Compressed Layouts for PUFs."
Abstract
Society continues to depend on electronics for everything from smart systems in our homes to cellphones and tablets, which are more powerful today than many desktop computers that are still in use [1]. With increased consumption of these electronic products comes an increase in problems, such as counterfeit integrated circuits being sold as genuine integrated circuits. This is just one of many problems that corporations and end users are having to deal with in this digital age.
Software security has been accepted as a problem by both the media and general public, but only recently has hardware security begun to come out as a problem as important, if not more important, than software security. When there is an issue with software, a software patch can be released to fix this issue. Due to the nature of hardware, this is not possible with hardware security problems. Often times, frrmware can be updated to partially address the problem, as in the case with the Intel and AMD processor flaws in the news recently [2]. However, there are many times that the only solution is to take the hardware system offline and replace the questionable components.
Hardware security researchers have been trying to fmd ways to use security circuits in hardware design to help combat this problem. One type of security circuit being researched and used today is called a Physically Unclonable Circuit (PUF). PUF circuits can be added onto an existing integrated circuit design, interrogated at fabrication for challenge-response pairs which are stored in a database, then checked against that database at any time in the lifecycle of that integrated circuit [3].
This research contributes to PUF security circuits by showing how different ways of laying out a circuit design on an integrated circuit can change the performance of the circuit, as well as if PUF circuits designed on SiGe BiCMOS can be used in the same way that PUF circuits fabricated using standard CMOS processes are used today.

Lilian Casias [Spring 2019]
03-20-2019
Biography
Lilian Casias defended her PhD thesis on Wednesday, March 20 at 10 am at the CHTM building. Dr. Ganesh Balakrishnan served as her committee chair. The title of Ms. Casias' dissertation is, "Transport in Mid-Wavelength Infrared (MWIR) p- and n- type InAsSb and InAs/InAsSb Type-II Strained Layer Superlattices (T2SLs) for infrared detection."
Abstract
III-V materials such as InAsSb ternaries and InAs/InAsSb Type-II Strained Layer Superlattices (T2SLs) have significant potential for infrared (IR) detector applications, including space-based detection, when utilized in a unipolar barrier detector architecture (nBn). However, recent studies revealed the quantum efficiency in nBn detectors degrades significantly faster from proton-irradiation induced displacement damage as compared to HgCdTe photodiodes. Improving the quantum efficiency radiation-tolerance is theoretically possible by enhancing vertical hole mobility and thereby the vertical hole diffusion length. The vertical hole mobility of T2SLs materials differs significantly from the lateral mobility and measuring it is much less straightforward.
In order to tackle vertical transport, in-plane or lateral transport must be better understood. There are added challenges to determining the in-plane bulk carrier concentration in narrow bandgap materials due to the potential for electron accumulation at the surface of the material and at its interface with the layer grown directly below it. Electron accumulation layers form high conductance electron channels that can dominate both resistivity and Hall-effect transport measurements. Therefore, to correctly determine the in-plane bulk concentration and mobility, temperature- and magnetic-field-dependent transport measurements in conjunction with Multi-Carrier Fit (MCF) analysis were utilized on a series of p-doped InAs0.91Sb0.09 samples on GaSb substrates. The samples are etched to different thicknesses and variable-field measurements are utilized to assist in confirming whether a carrier species represents bulk, interface or surface conduction.
Secondly, n-type temperature- and magnetic-field dependent measurements on InAsSb and InAs/InAsSb T2SLs materials were performed to extract the in-plane transport properties for all the carriers present in each sample under two different doping concentrations (undoped and Silicon-doped). Lastly, substrate-removed, metal-semiconductor-metal (MSM) devices were fabricated to attempt vertical measurements, while standard van der Pauw structures were used for in-plane measurements. The MSM processing serves as a potential fabrication technique to measure vertical transport, that can be improved in the future. The goal of this dissertation is to accurately determine the lateral and vertical transport properties in the presence of multiple carrier species, Multi-Carrier Fit (MCF) and High-Resolution Mobility Spectrum Analysis (HR-MSA) were employed.

Eli Garduno [Spring 2019]
09-06-2018
Biography
Eli Garduno defended his PhD thesis on Thursday Sept. 6, 2018 at 10 am in the CHTM Building. Dr. Ganesh Balakrishnan served as his committee chair. The title of Mr. Garduno's dissertation is, "Noise and Gain Characterization of Interband Cascade Infrared Photodetectors."
Abstract
Infrared (IR) detectors are an enabling technology for a broad and growing list of applications including gas detection, night vision, and space-based missile warning. There are ongoing efforts in IR detector research to explore the potential of new material systems and energy band structures in addition to continuously improving their sensitivity through increasing their quantum efficiency and lowering their dark current and noise. This dissertation examines an emerging class of IR detectors known as Interband Cascade Infrared Photodetectors (ICIPs).
ICIPs contain multiple regions to facilitate the collection of photogenerated electrons and to limit unwanted dark current. Theory regarding their performance also indicates that multi-stage ICIPs may have lower noise than single-stage ICIPs and may provide improved detectivity in cases where the absorption coefficient of a material system is small and/ or where the diffusion length in the material is short or degraded.
In this work, four long-wavelength infrared ICIP devices with one, four, six, and eight stages were characterized at varying temperatures from 80 to 300 K and at biases up to one volt in both forward and reverse polarities. Noise spectra were collected on the four devices and show significant 1 / f noise that prevented direct measurement of the ICIP noise gain. The 1/ f noise in the ICIPs was linked to generation-recombination current. The devices were found to cause circuit instability when operated in bias regions with negative differential conductance (NDC) due to bias-dependent resonant tunneling. Additionally, bias-dependent photocurrent gain was observed using illumination of the devices with 632 nm and 1550 nm lasers which peaked near the NDC regions. This photocurrent gain was experimentally shown to be caused by current-mismatch between device stages, verifying theories regarding its origin.

Nicholas Tarasenko [Spring 2019]
02-21-2018
Biography
Nicholas Tarasenko defended his PhD thesis on Thursday, February 21, 2019 at 1 pm in room 118 of the ECE Building. Dr. Christos Christodoulou served as his committee chair. The title of Mr. Tarasenko's dissertation is, "DESIGN AND IMPLEMENTATION OF A 72 & 84 GHZ TERRESTRIAL PROPAGATION EXPERIMENT; EXPLOITATION OF NEXRAD DATA TO STATISTICALLY ESTIMATE RAIN ATTENUATION AT 72 GHZ."
Abstract
The wireless communication sector is rapidly approaching network capacities as a direct result of increasing mobile broadband data demands. In response, the Federal Communications Commission allocated 71-76 GHz “V-band” and 81-86 GHz “W-band” for terrestrial and satellite broadcasting services. Movement by the telecommunication industry towards W/V-band operations is encumbered by a lack of validated and verified propagation models, specifically models to predict attenuation due to rain. Additionally, there is insufficient data available at W/V-bands to develop or test propagation models. The first aim of this study was the successful installation and operation of a terrestrial link to collect propagation data at W/V-band frequencies. In September 2015, the University of New Mexico, in collaboration with the Air Force Research Laboratory’s Space Vehicle Directorate, NASA’s Glenn Research Center and industry partners including (ACME, Applied Technology Associates, and Quinstar Technologies, Inc.) established the W/V-band Terrestrial Link Experiment (WTLE). WTLE was installed in the Albuquerque metro area with persistent tonal transmissions at 72 GHz and 84 GHz on a 23.5 km slanted path.
The second aim of this study was the utilization of the National Weather Service’s Next Generation Weather Radar (NEXRAD) system data to statistically estimate attenuation due to rain at 72 GHz. NEXRAD data provides a distributed sense of rain rates along WTLE’s path and alleviates challenges associated with instrumenting the 23.5 km link. Furthermore, NEXRAD data alleviates the need to develop complicated routines using in-situ meteorological measurements to estimate the size of the rain cell affecting the link. Non-linear regression techniques were applied on 2017 monsoon season data to obtain rain rate power law model coefficients. Testing of these coefficients was conducted on 2018 monsoon season data with satisfactory results. The techniques employed in this analysis represent a significant advancement in the ability to predict attenuation due to rain at 72 GHz for terrestrial links by enabling the use of historical archives of publicly available National Weather Service NEXRAD data. The technique has promising potential for estimation of path attenuation due to rain for links other than WTLE because of the vast nationwide coverage provided by NEXRAD systems.